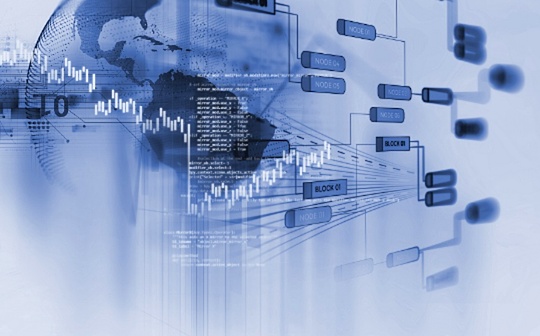
Author: YBB Capital Zeke
Preface
On February 16, OpenAI announced the latest text-controlled video generation diffusion model “Sora”, which demonstrates another milestone in generative AI through high-quality generation of videos of a wide range of visual data types covered by multiple segments.Unlike Pika, AI video generation tools are still in the state of generating videos for several seconds with multiple images, Sora has achieved scalable video generation by training in the compressed latent space of video and images and decomposing them into space-time position patches..In addition, the model also reflects the ability to simulate the physical world and the digital world. The final 60-second demo is not an exaggeration to say that it is a “general simulator of the physical world”.
In terms of construction methods, Sora continued the previous technical path of the GPT model “source data-Transformer-Diffusion-emergence”, which means that its development and maturity also require computing power as an engine, and since the amount of data required for video training is much larger than that of textThe amount of data trained will further increase the demand for computing power.However, we have already discussed the importance of computing power in the AI era in the early article “Popular Track Preview: Decentralized Computing Power Market”, and with the recent rising popularity of AI, there are already a large number of computing power projects on the market.It is beginning to emerge, and other Depin projects (storage, computing power, etc.) that have benefited passively have also ushered in a surge.So in addition to Depin, what kind of sparks can the interweaving of Web3 and AI collide?What other opportunities does this track contain?The main purpose of this article is to update and complete past articles, and to think about the possibilities of Web3 in the AI era.
Three major directions in the history of AI development
Artificial Intelligence is an emerging science and technology designed to simulate, expand and enhance human intelligence.Since its birth in the 1950s and 1960s, artificial intelligence has become an important technology to promote changes in social life and all walks of life after more than half a century of development.In this process, the interweaving development of the three research directions of symbolism, connectivity and behaviorism has become the cornerstone of the rapid development of AI today.
Symbolism
Also known as logicism or ruleism, it believes that it is feasible to simulate human intelligence by processing symbols.This method uses symbols to represent and operate objects, concepts and their interrelationships within the problem area, and uses logical reasoning to solve problems, especially in expert systems and knowledge representations.The core view of symbolism is that intelligent behavior can be achieved through operation and logical reasoning of symbols, where symbols represent a high degree of abstraction of the real world;
Connectionism
Or neural network methods, designed to achieve intelligence by mimicking the structure and functions of the human brain.This method enables learning by building a network of numerous simple processing units (like neurons) and adjusting the strength of connection between these units (like synapses).Connectionism emphasizes the ability to learn and generalize from data, and is particularly suitable for pattern recognition, classification and continuous input and output mapping problems.Deep learning, as the development of connectivism, has made breakthroughs in areas such as image recognition, speech recognition and natural language processing;
Behaviorism
Behaviorism is closely related to the research of bionic robotics and autonomous intelligent systems, emphasizing that agents can learn through interaction with the environment.Unlike the first two, behaviorism does not focus on simulating internal representations or thinking processes, but rather achieves adaptive behavior through a cycle of perception and action.Behaviorism believes that intelligence is manifested through dynamic interaction and learning with the environment, which is particularly effective when applied to mobile robots and adaptive control systems that require action in complex and unpredictable environments.
Although there are essential differences in these three research directions, they can also interact and integrate in actual AI research and application to jointly promote the development of the AI field.
AIGC principle overview
Artificial Intelligence Generated Content (AIGC), which is currently undergoing explosive development, is an evolution and application of connectivityism. AIGC can imitate human creativity to generate novel content.These models are trained using large datasets and deep learning algorithms to learn the underlying structures, relationships, and patterns that exist in the data.Generate novel and unique output results based on user input prompts, including images, videos, codes, music, design, translations, question answers and text.The current AIGC basically consists of three elements: deep learning (DL), big data, and large-scale computing power.
Deep Learning
Deep learning is a subfield of machine learning (ML), and deep learning algorithms are neural networks modeled according to the human brain.For example, the human brain contains millions of interrelated neurons that work together to learn and process information.Similarly, deep learning neural networks (or artificial neural networks) are composed of multi-layered artificial neurons that work together inside a computer.Artificial neurons are software modules called nodes that use mathematical calculations to process data.Artificial neural networks are deep learning algorithms that use these nodes to solve complex problems.
Dividing neural networks from a hierarchy can be divided into input layer, hidden layer, and output layer, and parameters are connected between different layers.
-
Input Layer: The input layer is the first layer of the neural network, which is responsible for receiving external input data.Each neuron in the input layer corresponds to a feature of the input data.For example, when processing image data, each neuron may correspond to a pixel value of the image;
-
Hidden Layer: The input layer processes the data and passes it to a further layer in the neural network.These hidden layers process information at different levels and adjust their behavior when receiving new information.Deep learning networks have hundreds of hidden layers that can be used to analyze problems from multiple different angles.For example, if you get an image of an unknown animal that must be classified, you can compare it with an animal you already know.For example, we can judge what animal this is based on the shape of the ear, the number of legs, and the size of the pupils.Hidden layers in deep neural networks work in the same way.If a deep learning algorithm tries to classify animal images, each of its hidden layers processes different features of the animal and attempts to accurately classify them;
-
Output layer: The output layer is the last layer of the neural network, which is responsible for generating the output of the network.Each neuron in the output layer represents a possible output category or value.For example, in a classification problem, each output layer neuron may correspond to a category, while in a regression problem, the output layer may have only one neuron, whose value represents the prediction result;
-
parameter: In neural networks, the connections between different layers are represented by weights and biases parameters, which are optimized during training to enable the network to accurately identify patterns in the data and make predictions.The increase in parameters can increase the model capacity of the neural network, i.e. the ability of the model to learn and represent complex patterns in the data.But the corresponding increase in parameters will increase the demand for computing power.
Big Data
For effective training, neural networks usually require large, diverse, high-quality and multi-source data.It is the basis for machine learning model training and verification.By analyzing big data, machine learning models can learn patterns and relationships in the data to make predictions or classifications.
Large-scale computing power
The multi-layer complex structure of neural networks, a large number of parameters, big data processing requirements, iterative training methods (in the training stage, the model needs to iterate repeatedly, and during the training process, it is necessary to propagate and backpropagate each layer of calculation, including activation functionsThe calculation of the loss function, the calculation of gradient and the update of weights, the requirements of high-precision calculation, parallel computing power, optimization and regularization technology, and model evaluation and verification processes have led to their demand for high computing power.
Sora
As OpenAI’s latest video generation AI model, Sora represents a huge advance in artificial intelligence’s ability to process and understand diverse visual data.By adopting video compression network and space time patch technology, Sora can convert massive visual data shot from different devices around the world into a unified form of expression, thus achieving efficient processing and understanding of complex visual content.Relying on the text-conditioned Diffusion model, Sora can generate highly matched videos or pictures based on text prompts, showing extremely high creativity and adaptability.
However, despite Sora’s breakthroughs in video generation and simulating real-world interactions, it still faces some limitations, including the accuracy of physical world simulations, the consistency of long video generation, the understanding of complex text instructions, and the training and generation efficiency.In essence, Sora continues the old technical path of “big data-Transformer-Diffusion-emergence” through OpenAI’s monopoly computing power and first-mover advantage, and has achieved a violent aesthetic. Other AI companies still have the technology curve.Possibility of overtaking.
Although Sora has little to do with blockchain, I personally think it will take the next one or two years.Because Sora’s influence will force other high-quality AI generation tools to emerge and develop rapidly, and will radiate to multiple tracks such as GameFi, social networking, creative platforms, and Depin in Web3, it is necessary to have a general understanding of Sora.How AI in the future will be effectively combined with Web3 may be a key point that we need to think about.
Four major paths to AI x Web3
As mentioned above, we can know that there are actually only three underlying bases required for generative AI: algorithms, data, and computing power. On the other hand, from the perspective of generalizability and generational effect, AI is a tool to subvert production methods.The biggest role of blockchain is two: reconstructing production relations and decentralization.So I personally think there are four ways to generate a collision between the two:
Decentralized computing power
Since we have written related articles in the past, the main purpose of this paragraph is to update the recent situation of the computing power track.When it comes to AI, computing power is always a difficult link to bypass.The great demand for computing power of AI is already unimaginable after Sora was born.Recently, during the 2024 World Economic Forum in Davos, Switzerland, OpenAI CEO Ultraman said bluntly that computing power and energy are the biggest shackles at this stage, and the importance of the two in the future will even be equivalent to currency..On February 10, Ultraman Sam released an extremely amazing plan to raise 7 trillion US dollars (equivalent to 40% of China’s national GDP in 23 years) to rewrite the current global semiconductor industry structure.Founded a chip empire.When writing articles related to computing power, my imagination is still limited to national blockade and giants monopolize it. It is really crazy for a company to want to control the global semiconductor industry.
Therefore, the importance of decentralized computing power is naturally self-evident. The characteristics of blockchain can indeed solve the problem of extremely monopoly computing power at present, as well as the expensive purchase of dedicated GPUs.From the perspective of AI, the use of computing power can be divided into two directions: reasoning and training. There are still very few projects focusing on training at present. From the need to combine neural network design with decentralized networks to super-hardwareHigh demand is destined to be a direction with extremely high threshold and extremely difficult to implement.Reasoning is relatively simple. On the one hand, it is not complicated in decentralized network design, and on the other hand, the hardware and bandwidth requirements are relatively low, which is currently the mainstream direction.
The imagination space of the centralized computing power market is huge, and it is often linked to the keyword “trillion-level”. It is also the most likely topic to be frequently hyped in the AI era.However, judging from the large number of projects that have emerged recently, most of them are still being on the shelves and taking advantage of the popularity.Always hold high the correct banner of decentralization, but keep silent about the inefficiency of decentralized networks.And there is a high degree of homogeneity in design, and a large number of projects are very similar (one-click L2 plus mining design), which may eventually lead to a mess. It is really difficult to get a piece of the traditional AI track in such a situation.
Algorithm and model collaboration system
Machine learning algorithms refer to these algorithms being able to learn laws and patterns from data and make predictions or decisions based on them.Algorithms are technology-intensive because their design and optimization require deep expertise and technological innovation.Algorithms are at the heart of training AI models, which define how data is translated into useful insights or decisions.More common generative AI algorithms such as Generative Adversarial Networks (GANs), Variational Autoencoders (VAEs), and Transformers. Each algorithm is for a specific field (such as painting, language recognition, translation, and video generation.) or purpose is born, and then a dedicated AI model is trained through algorithms.
So many algorithms and models have their own advantages. Can we integrate them into a model that can be literary and martial?Bittensor, which has been popular recently, is the leader in this direction. Through mining incentives, different AI models and algorithms can collaborate and learn from each other, thereby creating a more efficient and versatile AI model.Commune AI (code collaboration) and other aspects are also mainly based on this direction. However, algorithms and models are their own magic weapons for today’s AI companies and will not be borrowed accidentally.
Therefore, the narrative of the AI collaboration ecosystem is novel and interesting. The collaboration ecosystem takes advantage of the advantages of blockchain to integrate the disadvantages of AI algorithm islands, but it is still unknown whether it can create corresponding value.After all, the closed-source algorithms and models of leading AI companies have very strong capabilities in updates, iterations and integration. For example, OpenAI has been developing for less than two years and has been iterating from early text generation models to multi-field generation models. Bittensor and other projects have been in models and algorithms.The area you are targeting may have to find another way.
Decentralized big data
From a simple point of view, using private data to feed AI and tag data is a very consistent direction with blockchain. You only need to pay attention to how to prevent spam data and evil, and also enable FIL and AR in data storage.Depin projects benefit.From a complex perspective, using blockchain data for machine learning (ML) to solve the accessibility of blockchain data is also an interesting direction (one of Giza’s exploration directions).
In theory, blockchain data can be accessed at any time, reflecting the status of the entire blockchain.But for those outside the blockchain ecosystem, it is not easy to obtain these huge amounts of data.To fully store a blockchain requires rich expertise and a large amount of specialized hardware resources.To overcome the challenge of accessing blockchain data, several solutions have emerged in the industry.For example, RPC providers access nodes through APIs, while indexing services make data extraction possible through SQL and GraphQL, both of which play a key role in solving problems.However, these methods have limitations.RPC services are not suitable for high-density usage scenarios that require large amounts of data queries and often cannot meet the needs.Meanwhile, although indexing services provide a more structured way to retrieve data, the complexity of the Web3 protocol makes it extremely difficult to build efficient queries, sometimes requiring hundreds or even thousands of lines of complex code.This complexity is a huge obstacle for general data practitioners and those who don’t have a deep understanding of Web3 details.The cumulative effect of these limitations highlights the need for an easier way to access and utilize blockchain data that can facilitate wider application and innovation in the field.
Then, through ZKML (zero knowledge proof machine learning, reducing the burden on the chain of machine learning) combined with high-quality blockchain data, it may be possible to create data sets that solve the accessibility of blockchain, while AI can significantly reduce blockchain.The threshold for data accessibility, so over time, developers, researchers and enthusiasts in the ML field will be able to access more high-quality, relevant data sets for building effective and innovative solutions.
AI empowers Dapp
Since ChatGPT3 became popular in 23 years, AI empowering Dapps has become a very common direction.Generative AI with extremely widespread utility can be accessed through APIs, thereby simplifying and intelligently analyzing data platforms, trading robots, blockchain encyclopedia and other applications.On the other hand, you can also play as a chatbot (such as Myshell) or AI companion (Sleepless AI), and even create NPCs in chain games through generative AI.However, due to the low technical barriers, most of them are fine-tuned after accessing an API, and the combination with the project itself is not perfect, so it is rarely mentioned.
But after Sora arrives, I personally believe that the direction of AI empowering GameFi (including the metaverse) and creative platforms will be the focus of the next focus.Because of the bottom-up nature of the Web3 field, it is definitely difficult to produce products that compete with traditional games or creative companies, and the emergence of Sora is likely to break this dilemma (maybe only two to three years).Judging from Sora’s Demo, it already has the potential to compete with micro-short drama companies. Web3’s active community culture can also produce a large number of interesting Idea. When the restrictions are only imagination, the bottom-up industry andThe barriers between top-down traditional industries will be broken.
Conclusion
With the continuous advancement of generative AI tools, we will experience more epoch-making “iPhone moments” in the future.Although many people sneer at the combination of AI and Web3, I actually think most of the current direction is not a problem, and there are actually only three pain points that need to be solved, namely necessity, efficiency, and fit.Although the integration of the two is in the exploration stage, it does not prevent this track from becoming the mainstream of the next bull market.
Always maintaining enough curiosity and acceptance of new things is a necessary mentality for us. Historically, the transformation of cars replacing carriages has become a foregone conclusion. Just like the inscriptions and the NFTs in the past, they hold too many prejudices.It will only miss the opportunity.