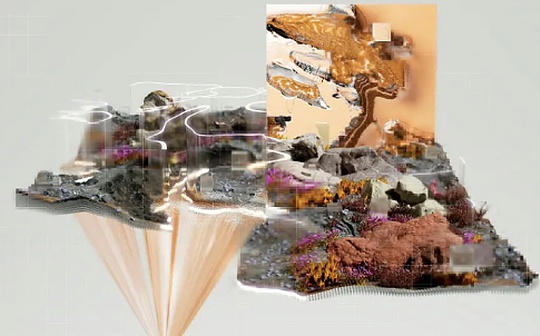
Author: Zeke, YBB Capital; Translation: 0xjs@作 作 作 作 作
Foreword
On February 16, OpenAI announced the launch of the latest Wensheng video -generating diffusion model called “Sora”. With its ability to generate high -quality video on various visual data types, it marks another milestone of the generation AI.Unlike PIKA and other AI video generating tools that generate several seconds of video from multiple images, SORA trains in the compressed potential space of video and images to decompose them into space -time patch to generate scalable videos.In addition, the model shows the ability of simulated physical and digital worlds, and its 60 -second demonstration is described as “general simulator of the physical world”.
Sora continues the technical path of the previous GPT model “Source Data-TRANSFORMER-DIFFUSION-EMERGENCE”, indicating that its development maturity also depends on computing power.Given that the amount of data required for video training is larger than text, its demand for computing power is expected to increase further.However, as we discussed in our previous article “Popularization of Potential Industry: Decentralization Becetic Market”, the importance of computing power in the AI era has been discussed. With the increasing popularity of AI, many computing power projects should be at the time of attempt to be at the time of attempts.Birth, benefiting the DEPIN project (storage, computing power, etc.), their value has increased.In addition to DEPIN, this article aims to update and improve past discussions, and think about the sparks that may be produced by Web3 and AI and the opportunities in the track in the AI era.
The three directions of AI development
AI is an emerging science and technology that aims to simulate, extend and enhance human intelligence.Since the birth of the 1950s and the 1960s, AI has developed for more than half a century, and has now become a key technology to promote social life and various industries.In the process, the intertwined development of the three major research directions of symbolism, connectionism, and behavioralism has laid the foundation for the rapid development of artificial intelligence today.
Symbolism
Symbolism is also known as logicalism or rules -based reasoning. It is considered that it is feasible to simulate human intelligence through symbolic processing.This method uses symbols to represent the objects, concepts and relationships in the problem domain, and use logical reasoning to solve the problem.Symbolism has achieved great success, especially in terms of expert systems and knowledge.The core idea of symbolism is that intelligent behavior can be achieved through symbolic manipulation and logical reasoning. The symbols represent the high -level abstraction of the real world.
Associatedism
Or it is called neural network method, to realize intelligence by imitating the structure and functions of the human brain.This method builds a network composed of many simple processing units (similar to neurons), and adjusts the connection intensity between these units (similar to the synapses) to promote learning.Connectism emphasizes the ability to learn and generalize from data, so that it is particularly suitable for mode recognition, classification, and continuous input and output mapping problems.As the evolution of connectionism, deep learning has made breakthroughs in the fields of image recognition, voice recognition, and natural language processing.
Behavioralism
Behaviorism is closely related to the research of bionic robots and autonomous intelligent systems, emphasizing that intelligence can learn through interaction with the environment.Unlike the previous two, behavioralism does not focus on simulating internal characteristics or thinking processes, but to achieve adaptive behavior through the cycle of perception and action.Behaviorism believes that intelligence is reflected through dynamic interaction and learning with the environment, which makes it particularly effective for mobile robots and adaptive control systems running in a complex and unpredictable environment.
Although there are fundamental differences in these three research directions, they can interact and integrate with each other in the actual research and application of AI to jointly promote the development of artificial intelligence.
AIGC principle
AIGC’s explosive development field represents the evolution and application of connectionism, and can generate novel content by imitating human creativity.These models use large datasets and deep learning algorithms for training, and the underlying structure, relationships and patterns in learning data.According to the user’s prompts, they generate unique outputs, including images, videos, code, music, design, translation, question answers and texts.At present, AIGC is basically composed of three elements: deep learning, big data and massive computing capabilities.
Deep learning
Deep learning is a sub -field of machine learning, which uses algorithms that imitate human neural networks.For example, the human brain consists of millions of interrelated neurons, and they work together to learn and process information.Similarly, deep learning neural networks (or artificial neural networks) consist of multi -layer artificial neurons that work together in computers.These artificial neurons (referred to as nodes) use mathematical calculations to process data.Artificial neural networks use these nodes to solve complex problems through deep learning algorithms.
The neural network is divided into layers: input layers, hidden layers and output layers, and parameters are connected to different layers.
Enter layer:The first layer of neural network is responsible for receiving external input data.Each neuron in the input layer corresponds to a feature of the input data.For example, when processing image data, each neuron may correspond to a pixel value of the image.
Hidden layer:The input layer processs the data and is further passed to the network.These hidden layers process information at different levels and adjust their behavior when receiving new information.There are hundreds of hidden layers in deep learning networks, which can analyze problems from multiple angles.For example, when an image of an unknown animal that needs to be classified, you can compare it with the animals you already know by checking the shape of the ears, the number of legs, and the size of the pupil.The hidden layer in the deep neural network works in a similar way.If deep learning algorithms try to classify animal images, each hidden layer will handle different characteristics of animals and try to accurately classify it.
Output layer:The last layer of the neural network is responsible for generating the output of the network.Each neuron in the output layer represents a possible output category or value.For example, in the classification problem, each output layer neuron may correspond to one category, and in the regression problem, the output layer may have only one neuron, and its value represents the predictive result.
parameter:In neural networks, the connections between different layers are represented by weights and deviations that they are optimized during the training process so that the network can accurately identify the patterns in the data and make predictions.Increasing parameters can enhance the ability of the neural network, that is, the ability to learn and represent complex mode in the data.However, this also increases the demand for computing power.
Big data
In order to conduct effective training, neural networks usually need a large, diverse, high -quality and multi -source data.It constitutes the basis for training and verification machine learning models.By analyzing big data, machine learning models can learn the patterns and relationships in the data, so as to achieve prediction or classification.
Massive computing power
The multi -layered structure of neural networks is complicated, there are many parameters, big data processing requirements, iterative training methods (the model needs to be repeatedly iterated during training, involving the forward and backward transmission calculations of each layer, including activation function calculation, loss function calculation, gradient levelCalculation and weight renewal), high -precision computing requirements, parallel computing capabilities, optimization and regularization technologies, and model evaluation and verification processes together lead to high computing power requirements.
Sora
As the latest video generating AI model of OpenAI, Sora represents a major progress of artificial intelligence processing and understanding of diversified visual data.By using video compression network and space -time patch technology, Sora can convert massive visual data captured by different devices worldwide into a unified representation form, thereby achieving efficient processing and understanding of complex visual content.Using text conditions to diffuse models, Sora can generate videos or images that are highly matched with text prompts, showing high creativity and adaptability.
However, although Sora has made breakthroughs in the interaction of video generation and simulation in real world, it still faces some limitations, including the accuracy of the physical world simulation, the consistency of the growth video, the complex text instructions, and the training and generation of generationefficiency.In essence, Sora continues the old technical path of the “Big Data-TRANSFORMER-Diffusion-Emergeence” through OpenAI’s monopoly computing power and first-mover advantages, and realizes a brute aesthetics.Other artificial intelligence companies still have the potential to surpass through technological innovation.
Although the relationship between SORA and the blockchain is not great, I believe that in the next year or two, due to the influence of Sora, other high -quality AI generation tools will appear and develop rapidly, impact, DEPIN, etc.Therefore, it is necessary to have a general understanding of Sora. How to effectively combine AI with Web3 in the future is a key consideration.
Four ways of AI X Web3 fusion
As discussed earlier, we can understand that the basic elements required by the generation AI are essentially three: algorithms, data and computing power.On the other hand, considering its universality and output effect, AI is a tool that completely changes the production method.At the same time, the biggest impact of the blockchain is dual: reorganization of production relations and decentralization.
Therefore, I think the collision of these two technologies can produce the following four paths:
Decentralization
As mentioned earlier, this section aims to update the status of computing capabilities.When it comes to AI, computing power is an indispensable aspect.The emergence of Sora made the unimaginable AI demand for computing power.Recently, during the Davos World Economic Forum in Switzerland in 2024, OPENAI CEO Sam Altman publicly stated that computing power and energy are the biggest constraints at present, implying that their future importance may even be equivalent to currency.Subsequently, on February 10, Sam Altman announced a shocking plan on Twitter that will raise 7 trillion US dollars (equivalent to 40%of China’s GDP in 2023) to completely reform the current global semiconductor industry.Create a semiconductor empire.My previous thinking about computing power is limited to national blockade and corporate monopoly; the idea of a company to dominate the global semiconductor industry is indeed crazy.
Therefore, the importance of decentralized computing power is self -evident.The characteristics of the blockchain can indeed solve the problem of extreme monopoly at the current computing power, as well as the expensive cost related to obtaining a dedicated GPU.From the perspective of AI requirements, the use of computing power can be divided into two directions: reasoning and training.There are very few projects focusing on training, because decentralized networks need to integrate neural network design, and the hardware requirements are extremely high. It is a direction with higher thresholds and difficult implementation.In contrast, the reasoning is relatively simple, because the decentralized network design is not so complicated, and the requirements for hardware and bandwidth are also low, which is a more mainstream direction.
The centralized computing power market has a wide range of imagination, often linked with the “trillion levels” keywords, which is also the easiest topic of the AI era.However, looking at the many projects that have appeared recently, most of them seem to use trend to consider unexpected attempts.They often raise the flag of decentralization, but avoid discussing the inefficiency of decentralized networks.In addition, the degree of homogeneity of design is very high. Many projects are very similar (one -click L2 plus mining design), which may eventually lead to failure, and it is difficult to occupy a place in traditional AI competitions.
Algorithm and model collaborative system
Machine learning algorithms are algorithms that can learn modes and rules from the data and make predictions or decisions based on them.The algorithm is technology dense, because its design and optimization requires deep professional knowledge and technological innovation.Algorithms are the core of training artificial intelligence models, defining how to transform data into useful insights or decisions.Common generation of AI algorithms include generating confrontation networks (GAN) (GAN), transformer self -encoder (VAE), and Transformers. Each algorithm is designed for specific fields (such as painting, language recognition, translation, video generation) or purposeAnd then used to train specialized AI models.
So, there are so many algorithms and models, each of which is possible to integrate them into a common model?Bittersor is a recent project that has attracted much attention. It cooperates and learn from different AI models and algorithms to create more efficient and capable AI models, thereby leading this direction.Other projects focusing on this direction include Commune AI (code collaboration), but algorithms and models are strictly confidential for AI companies and are not easy to share.
Therefore, the narrative of the AI collaborative ecosystem is novel and interesting.The collaborative ecosystem uses the advantages of the blockchain to integrate the disadvantage of the isolated AI algorithm, but it remains to be observed whether it can create corresponding value.After all, the leading AI company with independent algorithms and models has strong updates, iterations and integration capabilities.For example, OPENAI has developed from early text generation models to multi -field generation models in less than two years.Projects like Bittersor may need to explore new paths in their models and algorithm target fields.
Decentralization big data
From a simple point of view, the use of privacy data to feed AI and annotation data is very consistent with blockchain technology. The main consideration is how to prevent junk data and malicious behaviors.In addition, data storage can benefit the DEPIN projects such as Fil and AR.From a more complicated perspective, using blockchain data for machine learning to solve the accessability of blockchain data is another interesting direction (one of GIZA exploration).
Theoretically, blockchain data can be accessed at any time, reflecting the state of the entire blockchain.However, for people outside the blockchain ecosystem, it is not easy to access these large amounts of data.Storing the entire blockchain requires rich professional knowledge and a large number of professional hardware resources.In order to overcome the challenges of access to the blockchain data, various solutions have appeared in the industry.For example, RPC providers provide node access through the API, and index services make data retrieval possible through SQL and GraphQL, which plays a vital role in solving this problem.However, these methods have their limitations.RPC services are not suitable for high -density cases that need a large amount of data query, and often cannot meet the needs.At the same time, although the index service provides a more structural data retrieval method, the complexity of the Web3 protocol makes it extremely difficult for structural efficient queries, and sometimes hundreds or even thousands of complex code are required.This complexity is a major obstacle for general data practitioners and those who have a limited understanding of Web3 details.These restricted cumulative effects highlight the need to access and use blockchain data that requires more access and use, which can promote wider application and innovation in the field.
Therefore, combining ZKML (zero -knowledge proof of machine learning, reducing the burden on machine learning on the chain) and high -quality blockchain data may create data sets that solve the accessability of blockchain data.AI can significantly reduce the access barrier of blockchain data.Over time, developers, researchers and machine learning enthusiasts can access more high -quality and related data sets to build effective and innovative solutions.
AI empowerment DAPP
Since the outbreak of ChatGPT3 in 2023, AI’s empowerment of DAPP has become a very common direction.Extensive application -based artificial intelligence can be integrated through API, thereby simplifying and intelligent data platforms, trading robots, blockchain encyclopedia, and other applications.On the other hand, it can also act as a chat robot (such as MyShell) or AI partner (Sleepless AI), and can even use generatory AI to create NPCs in the blockchain game.However, because the technical threshold is low, most of them are just adjustments after integrated API, and the integration of the project itself is not perfect, so it is rarely mentioned.
But with the arrival of Sora, I personally think that AI’s empowerment of Gamefi (including Yuan universe) and creative platforms will be the focus of the future.Given the bottom -up nature of the Web3 field, it is unlikely to produce products that can compete with traditional games or creative companies.However, the emergence of Sora may break this deadlock (maybe only two to three years).Judging from Sora’s demonstration, it has the potential to compete with short drama companies.The active community culture of the web3 can also give birth to a lot of interesting ideas. When the only limit is the imagination, the barriers between the bottom -up industry and the top -down traditional industry will be broken.
in conclusion
With the continuous development of generating artificial intelligence tools, we will witness more breakthrough “iPhone moments” in the future.Although people are skeptical of the integration of AI and Web3, I believe that the current direction is basically correct, and only three main pain points are required: necessity, efficiency, and fit.Although the integration of the two is still in the exploration stage, it does not prevent this path from becoming the mainstream of the next bull market.
Maintaining enough curiosity and openness of new things is our basic mentality.Historically, the transformation from a carriage to a car was instantly solved, as the inscriptions and the past NFT were shown.Holding too much prejudice will only cause missed opportunities.