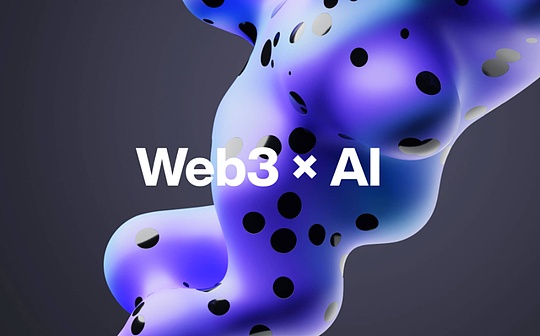
Introduction: The development of AI+Web3
In the past few years, the rapid development of artificial intelligence (AI) and Web3 technologies has attracted widespread attention worldwide.As a technology that simulates and imitates human intelligence, AI has made major breakthroughs in the fields of face recognition, natural language processing, machine learning, etc.The rapid development of AI technology has brought tremendous changes and innovations to all walks of life.
The market size of the AI industry reached US$200 billion in 2023. Industry giants and outstanding players such as OpenAI, Character.AI, Midjourney have emerged like mushrooms after a rain, leading the AI craze.
At the same time, Web3, as an emerging network model, is gradually changing our understanding and use of the Internet.Based on decentralized blockchain technology, Web3 realizes data sharing and controllability, user autonomy and trust mechanisms through functions such as smart contracts, distributed storage and decentralized authentication.The core concept of Web3 is to liberate data from centralized authoritative organizations and give users the right to control the data and share data value.
Currently, the market value of the Web3 industry has reached 25 trillion. Whether it is Bitcoin, Ethereum, Solana, Uniswap, Stepn and other players in the application layer, new narratives and scenarios are emerging one after another, attracting more and more people to join the Web3 industry.
It is easy to find that the combination of AI and Web3 is an area that both builders and VCs in the East and the West are very concerned about. How to integrate the two well is a very worth exploring question.
This article will focus on the current development status of AI+Web3 and explore the potential value and impact of this integration.We will first introduce the basic concepts and features of AI and Web3, and then explore their relationship.We will then analyze the current status of AI+Web3 projects and discuss in depth the limitations and challenges they face.Through such research, we expect to provide valuable reference and insights to investors and practitioners in related industries.
How AI interacts with Web3
The development of AI and Web3 is like both sides of the balance. AI has brought about improvements in productivity, while Web3 has brought about changes in production relations.So what kind of sparks can AI and Web3 collide?Next, we will analyze the difficulties and improvement space faced by the AI and Web3 industries, and then discuss how each other can help solve these difficulties.
-
Dilemma and potential improvement space for the AI industry
-
Dilemma and potential improvement space for the Web3 industry
2.1 Dilemma faced by the AI industry
If you want to explore the difficulties faced by the AI industry, let’s first look at the essence of the AI industry.The core of the AI industry is inseparable from three elements: computing power, algorithms and data.
-
First of all, computing power: Computing power refers to the ability to perform large-scale calculations and processing.AI tasks often require processing large amounts of data and performing complex computations, such as training deep neural network models.High-intensity computing power can accelerate model training and inference processes and improve the performance and efficiency of AI systems.In recent years, with the development of hardware technologies, such as graphics processors (GPUs) and dedicated AI chips (such as TPUs), the improvement of computing power has played an important role in promoting the development of the AI industry.Nvidia, which has soared stocks in recent years, has occupied a large market share as a GPU provider and made high profits.
-
What is an algorithm: Algorithms are the core components of AI systems, and they are mathematical and statistical methods used to solve problems and implement tasks.AI algorithms can be divided into traditional machine learning algorithms and deep learning algorithms, among which deep learning algorithms have made major breakthroughs in recent years.The selection and design of algorithms are crucial to the performance and effectiveness of AI systems.Continuously improved and innovative algorithms can improve the accuracy, robustness and generalization capabilities of AI systems.Different algorithms will have different effects, so improving the algorithm is also crucial to the effect of completing tasks.
-
Why data is important: The core task of AI systems is to extract patterns and rules in data through learning and training.
Data is the basis for training and optimizing models. Through large-scale data samples, AI systems can learn more accurate and smarter models.Rich data sets can provide more comprehensive and diverse information, allowing models to be better generalized to unseen data, helping AI systems better understand and solve real-world problems.
After understanding the three core elements of AI, let’s take a look at the difficulties and challenges that AI encounters in these three aspects. First of all, in terms of computing power. AI tasks usually require a large amount of computing resources for model training and reasoning, especially forIn terms of deep learning models.However, acquiring and managing large-scale computing power is an expensive and complex challenge.The cost, energy consumption and maintenance of high-performance computing equipment are all issues.Especially for startups and individual developers, it can be difficult to obtain enough computing power.
In terms of algorithms, although deep learning algorithms have achieved great success in many areas, there are still some dilemmas and challenges.For example, training deep neural networks requires a large amount of data and computing resources, and for some tasks, the explanatory and interpretable model may be insufficient.In addition, the robustness and generalization ability of the algorithm are also an important issue, and the model’s performance on unseen data may be unstable.Among the many algorithms, how to find the best algorithm to provide the best services is a process that requires continuous exploration.
In terms of data, data is the driving force of AI, but obtaining high-quality and diverse data remains a challenge.Data in some areas may be difficult to obtain, such as sensitive health data in the medical field.In addition, the quality, accuracy and labeling of data are also problems, and incomplete or biased data can lead to wrong behavior or bias in the model.At the same time, protecting data privacy and security is also an important consideration.
In addition, there are problems such as interpretability and transparency, and the black box characteristics of the AI model are a public concern.For some applications, such as finance, healthcare, and justice, the decision-making process of the model needs to be interpretable and traceable, while existing deep learning models often lack transparency.Explaining the decision-making process of the model and providing trustworthy explanations remains a challenge.
In addition, the business model of many AI project entrepreneurship is not very clear, which also makes many AI entrepreneurs feel confused.
2.2 Dilemma faced by the Web3 industry
In the Web3 industry, there are currently many difficulties in different aspects that need to be solved, whether it is the data analysis of Web3, the poor user experience of Web3 products, or the problems of smart contract code vulnerabilities and hacker attacks,Lots of room for improvement.As a tool to improve productivity, AI also has a lot of potential room for development in these aspects.
First of all, the improvement of data analysis and prediction capabilities: the application of AI technology in data analysis and prediction has brought a huge impact to the Web3 industry.Through intelligent analysis and mining of AI algorithms, the Web3 platform can extract valuable information from massive data and make more accurate predictions and decisions.This is of great significance to risk assessment, market forecasting and asset management in the field of decentralized finance (DeFi).
In addition, improvements in user experience and personalized services can also be achieved: the application of AI technology enables the Web3 platform to provide better user experience and personalized services.Through the analysis and modeling of user data, the Web3 platform can provide users with personalized recommendations, customized services and intelligent interactive experience.This helps improve user engagement and satisfaction and promotes the development of the Web3 ecosystem. For example, many Web3 protocols are connected to AI tools such as ChatGPT to better serve users.
In terms of security and privacy protection, the application of AI also has a profound impact on the Web3 industry.AI technology can be used to detect and defend against cyber attacks, identify abnormal behaviors, and provide stronger security.At the same time, AI can also be used for data privacy protection, and through technologies such as data encryption and privacy computing, it can protect users’ personal information on the Web3 platform.In terms of smart contract auditing, due to possible vulnerabilities and security risks in the writing and auditing of smart contracts, AI technology can be used to automate contract audits and vulnerability detection to improve the security and reliability of contracts.
It can be seen that AI can participate and provide assistance in many aspects in the difficulties and potential improvement space facing the Web3 industry.
Analysis of the current status of AI+Web3 projects
Projects combining AI and Web3 mainly start from two major aspects, use blockchain technology to improve the performance of AI projects, and use AI technology to serve the improvement of Web3 projects.
A large number of projects have emerged to explore this path, including various projects such as Io.net, Gensyn, Ritual, etc. Next, this article will use AI to help web3 and Web3 to help AI different sub-competitions.Analysis of the current situation and development.
3.1 Web3 helps AI
3.1.1 Decentralized computing power
Since OpenAI launched ChatGPT at the end of 2022, it has triggered a wave of AI. Five days after its launch, the number of users reached 1 million, while Instagram spent about two and a half months to reach 1 million downloads.After that, Chatgpt made great efforts very rapidly, with the number of monthly active users reaching 100 million within two months. By November 2023, the number of weekly active users reached 100 million.With the advent of Chatgpt, the AI field has also rapidly exploded from a niche track to a highly-watched industry.
According to Trendforce’s report, ChatGPT requires 30,000 NVIDIA A100 GPUs to run, and the GPT-5 will require more orders of magnitude calculations in the future.This also opened an arms race between various AI companies. Only by mastering enough computing power can we be sure that there is enough power and advantages in the AI battle, and therefore the GPU shortage occurs.
Before the rise of AI, Nvidia, the largest provider of GPUs, had its customers focused on three major cloud services: AWS, Azure, and GCP.With the rise of artificial intelligence, a large number of new buyers emerged, including big tech companies Meta, Oracle, and other data platforms and AI startups, all joining the war to hoard GPUs to train AI models.Large tech companies such as Meta and Tesla have increased purchases of customized AI models and internal research.Basic model companies like Anthropic and data platforms like Snowflake and Databricks have also purchased more GPUs to help customers provide AI services.
As mentioned by Semi Analysis last year, “GPU rich and GPU poor”, a few companies have more than 20,000 A100/H100 GPUs, and team members can use 100 to 1,000 GPUs for projects.These companies are either cloud providers or self-built LLMs, including OpenAI, Google, Meta, Anthropic, Inflection, Tesla, Oracle, Mistral, etc.
However, most companies are GPU poor and can only struggle with a much smaller number of GPUs, spending a lot of time and energy on things that are more difficult to drive the development of the ecosystem.And this situation is not limited to startups.Some of the most well-known AI companies – Hugging Face, Databricks (MosaicML), Together and even Snowflake have less than 20K in number of A100/H100.These companies have world-class technical talents, but are limited by the supply of GPUs, and are at a disadvantage compared to large companies in the competition in artificial intelligence.
This shortage is not limited to the “GPU poor”. Even at the end of 2023, OpenAI, the leader of the AI track, had to turn off paid registration for several weeks because it could not obtain enough GPUs, while purchasing more GPU supplies.
It can be seen that with the rapid development of AI, there is a serious mismatch between the demand and supply sides of GPUs, and the problem of supply shortage is imminent.
In order to solve this problem, some Web3 project parties have begun to try to provide decentralized computing power services based on the technical characteristics of Web3, including Akash, Render, Gensyn, etc.What’s common about this type of project is that tokens are used to incentivize users to provide idle GPU computing power, becoming the supply side of computing power to provide AI customers with computing power.
The supply-side portrait can be mainly divided into three aspects: cloud service providers, cryptocurrency miners, and enterprises.
Cloud service providers include large cloud service providers (such as AWS, Azure, GCP) and GPU cloud service providers (such as Coreweave, Lambda, Crusoe, etc.), and users can resell the computing power of idle cloud service providers to earn revenue.Crypto miners As Ethereum switches from PoW to PoS, idle GPU computing power has also become an important potential supply side.In addition, large companies like Tesla and Meta that have purchased a large number of GPUs due to their strategic layout can also use idle GPU computing power as the supply side.
Currently, players on the track are roughly divided into two categories: one is to use decentralized computing power for AI reasoning, and the other is to use decentralized computing power for AI training.The former is such as Render (although it focuses on rendering, but can also be used as a providing AI computing power), Akash, Aethir, etc.; the latter is such as io.net (both inference and training can be supported), Gensyn, the biggest difference between the two is computing.The requirements for strength are different.
Let’s first talk about the former AI reasoning project. This type of project attracts users to participate in the provision of computing power through token incentives, and then provides computing power network services to the demand side, thus realizing the supply and demand matching of idle computing power.The introduction and analysis of such projects was mentioned in our research report on DePIN before Ryze Labs.Welcome to check it out.
The most core point is that through the token incentive mechanism, the project first attracts suppliers and then attracts users to use, thus realizing the project’s cold start and core operation mechanism, which can further expand and develop.Under this cycle, the supply side has more and more valuable token returns, and the demand side has cheaper and more cost-effective services. The token value of the project and the growth of both supply and demand participants remain consistent.The rise in token prices attracts more participants and speculators to participate and form a value capture.
Another type is the use of decentralized computing power for AI training, such as Gensyn, io.net (AI training and AI reasoning can both support it).In fact, there is not much essential difference between the operation logic of this type of project and the AI reasoning project. It still attracts the participation of the supply side through token incentives to provide computing power for use by the demand side.
Among them, io.net is a decentralized computing power network, currently has more than 500,000 GPUs, and has performed outstandingly in decentralized computing power projects. In addition, it has also integrated the computing power of Render and filecoin and has begun to develop continuously.Ecological project.
In addition, Gensyn realizes AI training through smart contracts that can promote task allocation and rewards for machine learning.As shown in the figure below, Gensyn’s machine learning training work costs about $0.4 per hour, which is much lower than the cost of AWS and GCP for more than $2.
Gensyn’s system includes four participants: submitter, executor, verifier and whistleblower.
-
Submitted by: The user is the consumer of the task, providing the tasks to be calculated, and paying for AI training tasks
-
Executor: The executor performs the task trained by the model and generates a proof of completing the task for verification by the verifier.
-
Verifier: Linking the nondeterministic training process to the deterministic linear calculation, comparing the executor’s proof to the expected threshold.
-
Whistleblower: Check the work of the validator, and raise questions to gain profit when it is discovered.
It can be seen that Gensyn hopes to become a super-large-scale, cost-effective computing protocol for global deep learning models.But looking at this track, why do most projects choose decentralized computing power for AI reasoning instead of training?
Here we also help friends who don’t understand AI training and reasoning introduce the differences between the two:
-
AI training: If we compare artificial intelligence to a student, then training is similar to providing artificial intelligence with a lot of knowledge, and examples can also be understood as data we often call it, and artificial intelligence learns from these knowledge examples.Since the nature of learning requires understanding and memory of a large amount of information, this process requires a lot of computing power and time.
-
AI reasoning: So what is reasoning?It can be understood as using the knowledge learned to solve problems or take exams. In the reasoning stage, artificial intelligence uses the knowledge learned to solve them, rather than engaging in new knowledge, so the calculation amount required in the reasoning process is smaller..
It can be seen that the computing power requirements of the two are quite different. The availability of decentralized computing power in AI inference and AI training will be analyzed in a more in-depth manner in the subsequent challenge chapters.
In addition, Ritual hopes to combine distributed networks with model creators to maintain decentralization and security.Its first product, Internet, allows smart contracts on the blockchain to access AI models off-chain, allowing such contracts to access AI in a way that maintains verification, decentralization and privacy.
The coordinator of the Internet is responsible for managing the behavior of nodes in the network and responding to computing requests issued by consumers.When a user uses the Internet, inference, proof and other work will be placed off the chain, the output results will be returned to the coordinator, and finally passed to the consumers on the chain through the contract.
In addition to decentralized computing power networks, there are also decentralized bandwidth networks such as Grass to improve the speed and efficiency of data transmission.In general, the emergence of decentralized computing power networks has provided a new possibility for the computing power supply side of AI, pushing AI to move forward in a further direction.
3.1.2 Decentralized algorithm model
As mentioned in Chapter 2, the three core elements of AI are computing power, algorithms and data.Since computing power can form a supply network through decentralization, can the algorithm have similar ideas to form an algorithm model supply network?
Before analyzing the track project, let us first understand the significance of the decentralized algorithm model. Many people will be curious. Since OpenAI already exists, why do we still need a decentralized algorithm network?
In essence, a decentralized algorithm network is a decentralized AI algorithm service market that links many different AI models. Each AI model has its own knowledge and skills. When users ask questions, the market will chooseFind the AI model that is best suited to answer questions to provide answers.Chat-GPT is an AI model developed by OpenAI, which can understand and produce texts similar to humans.
Simply put, ChatGPT is like a student with strong abilities to help solve different types of problems, while a decentralized algorithm network is like a school with many students to help solve problems. Although the student is very capable now,From a long-term perspective, schools that can recruit students from all over the world have great potential.
Currently, in the field of decentralized algorithm models, there are also some projects in their attempts and explorations. Next, the representative project Bittensor will be used as a case to help everyone understand the development of this subdivided field.
In Bittensor, the supply side of the algorithmic model (or miners) contribute their machine learning models to the network.These models can analyze data and provide insights.Model providers will receive cryptocurrency token TAO as a reward for their contributions.
To ensure the quality of answers to questions, Bittensor uses a unique consensus mechanism to ensure that the network agrees on the best answers.Multiple model miners provide answers when asked.The validator in the network then starts working, determines the best answer, and sends it back to the user.
Bittensor’s token TAO mainly plays two roles in the entire process. On the one hand, it is used as an algorithmic model to incentivize miners to contribute to the network, and on the other hand, users need to spend tokens to ask questions and let the network complete tasks.
Since Bittensor is decentralized, anyone with internet access can join the network, both as a user asking questions and as a miner providing answers.This allows more people to use powerful artificial intelligence.
In short, taking networks such as Bittensor as an example, the decentralized field of algorithmic modeling has the potential to create a more open and transparent situation in which artificial intelligence models can be trained, shared and utilized in a secure and decentralized way.In addition, there are decentralized algorithmic model networks like BasedAI trying similar things. Of course, the more interesting part is to protect the interactive data privacy of users and models through ZK, which will be further discussed in the fourth section.
As decentralized algorithmic model platforms evolve, they will enable small companies to compete with large organizations in using top AI tools, thus having a potentially significant impact on various industries.
3.1.3 Decentralized data collection
For the training of AI models, a large amount of data supply is essential.However, most web2 companies still take users’ data for themselves. For example, platforms such as X, Reddit, TikTok, Snapchat, Instagram, YouTube, etc. prohibit data collection for artificial intelligence training.It has become a big obstacle to the development of the AI industry.
However, on the other hand, some Web2 platforms sell user data to AI companies without sharing any profit to users.For example, Reddit and Google reached a $60 million deal to let Google train AI models on its posts.This leads to the data collection rights being occupied by big capital and big data parties, leading to the industry’s super-capital-intensive development.
Faced with this current situation, some projects combine Web3 with token incentives to achieve decentralized data collection.Taking PublicAI as an example, in PublicAI users can participate as two types of roles:
-
One category is the provider of AI data. Users can find valuable content on X. @PublicAI official and attach insights. Using #AI or #Web3 as classification tags, the content can be sent to the PublicAI data center to realize the data.collect.
-
Another category is data validators, where users can log in to the PublicAI data center and select the most valuable data for AI training to vote.
In return, users can obtain token incentives through these two types of contributions, thereby promoting a win-win relationship between data contributors and the development of the artificial intelligence industry.
In addition to projects like PublicAI that specializes in collecting data for AI training, many projects are also collecting decentralized data through token incentives. For example, Ocean collects user data through data tokenization to serve AI.Hivemapper collects map data through the user’s car camera, Dimo collects user’s car data, WiHi collects weather data, etc. These projects that collect data through decentralization are also the supply side of potential AI training, so fromBroadly speaking, it can also be included in the paradigm of Web3 to help AI.
3.1.4 ZK protects user privacy in AI
In addition to the advantages of decentralization, another very important thing brought by blockchain technology is zero-knowledge proof.Through zero-knowledge technology, privacy can be protected while verifying information.
In traditional machine learning, data usually needs to be centrally stored and processed, which may lead to the risk of data privacy breaches.On the other hand, methods that protect data privacy, such as data encryption or data de-identification, may limit the accuracy and performance of machine learning models.
Zero-knowledge proof technology can help face this dilemma and resolve the conflict between privacy protection and data sharing.
ZKML (Zero-Knowledge Machine Learning) allows training and inference of machine learning models without revealing raw data by using zero-knowledge proof technology.Zero-knowledge proof enables the characteristics of data and the results of the model to be proved correct without revealing the actual data content.
The core goal of ZKML is to achieve a balance between privacy protection and data sharing.It can be applied to various scenarios, such as medical and health data analysis, financial data analysis, and cross-organizational cooperation.By using ZKML, individuals can protect the privacy of their sensitive data while sharing data with others for broader insights and opportunities to collaborate without worrying about the risk of data privacy breaches.
The field is still in its early stages and most projects are still being explored. For example, BasedAI proposes a decentralized approach that seamlessly integrates FHE with LLM to maintain data confidentiality.Embed privacy at the heart of its distributed network infrastructure using the Zero Knowledge Large Language Model (ZK-LLM) to ensure user data remains private throughout the network operation.
Here we briefly explain what is fully homomorphic encryption (FHE).Fully homomorphic encryption is an encryption technology that can calculate data in an encrypted state without decryption.This means that various mathematical operations (such as addition, multiplication, etc.) performed on data encrypted using FHE can be performed while keeping the data encrypted and obtain the results obtained by performing the same operations on the original unencrypted data.This protects the privacy of user data.
In addition, in addition to the above four categories, in terms of Web3’s AI support, there are also blockchain projects like Cortex that support the execution of AI programs on the chain.Currently, executing machine learning programs on traditional blockchains faces a challenge, where virtual machines are extremely inefficient when running any non-complex machine learning model.Therefore, most people think it is impossible to run artificial intelligence on blockchains.Cortex Virtual Machine (CVM) uses GPUs to execute AI programs on the chain and is compatible with EVM.In other words, the Cortex chain can execute all Ethereum Dapps and incorporate AI machine learning into these Dapps on this basis.This enables the operation of machine learning models in a decentralized, immutable and transparent manner, as network consensus validates every step of AI reasoning.
3.2 AI helps web3
In the collision between AI and Web3, in addition to the help of Web3 to AI, AI’s help to the Web3 industry is also worthy of attention.The core contribution of artificial intelligence lies in the improvement of productivity, so there are many attempts in AI audit smart contracts, data analysis and prediction, personalized services, security and privacy protection.
3.2.1 Data analysis and forecasting
At present, many Web3 projects have begun to integrate existing AI services (such as ChatGPT) or develop their own services to provide data analysis and prediction services to Web3 users.It covers a wide range, including providing investment strategies through AI algorithms, on-chain analysis AI tools, price and market forecasts, etc.
For example, Pond uses AI graph algorithm to predict valuable alpha tokens in the future, providing investment assistance advice to users and institutions; and BullBear AI trains users based on their historical data, price line history and market trends to provide the most accurate informationTo support predict price trends, help users obtain profits and profits.
There are also investment competition platforms such as Numerai. Participants predict the stock market based on AI and large language models, use the platform to provide free high-quality data training models, and submit predictions every day.Numerai calculates how these predictions perform over the next month, and contestants can bet on NMR on the model and earn money based on the model’s performance.
In addition, on-chain data analysis platforms such as Arkham also combine AI for services.Arkham links blockchain addresses to entities such as exchanges, funds and giant whales, and presents users with key data and analytics of these entities to provide users with decision-making advantages.Part of its combination with AI is that Arkham Ultra uses algorithms to match addresses with real-world entities, developed by Arkham core contributors with the support of Palantir and the founders of OpenAI over three years.
3.2.2 Personalized Service
In Web2 projects, AI has many application scenarios in the fields of search and recommendation, serving users’ personalized needs.The same is true in Web3 projects. Many project parties optimize users’ user experience by integrating AI.
For example, Dune, a well-known data analysis platform, recently launched the Wand tool for writing SQL queries with the help of large language models.Through the Wand Create function, users can automatically generate SQL queries based on natural language problems, so that users who do not understand SQL can also search very easily.
In addition, some Web3 content platforms have also begun to integrate ChatGPT for content summary. For example, Web3 media platform Followin integrates ChatGPT to summarize the views and latest situations of a certain track; Web3 encyclopedia platform IQ.wiki is committed to becoming an online areaThe main source of all objective and high-quality knowledge related to blockchain technology and cryptocurrencies makes blockchain easier to discover and acquire on a global scale, and provides users with information they can trust. It also integrates GPT-4To summarize wiki articles; Kaito, a search engine based on LLM, is committed to becoming a Web3 search platform and changing the way Web3 obtains information.
In terms of creation, there are also projects like NFPrompt that reduce user creation costs.NFPrompt allows users to generate NFTs more easily through AI, thereby reducing the user’s creative costs and providing many personalized services in terms of creation.
3.2.3 AI Audit Smart Contract
In the Web3 field, auditing of smart contracts is also a very important task. Through AI, the audit of smart contract code can be more efficiently and accurately identified and found vulnerabilities in the code.
As Vitalik once mentioned, one of the biggest challenges facing the cryptocurrency space is the errors in our code.And one expected possibility is that artificial intelligence (AI) can significantly simplify the use of formal verification tools to prove that a set of codes satisfying specific attributes.If we can do this, it is possible that we have error-free SEK EVMs (such as Ethereum virtual machines).The more you reduce the number of errors, the more security the space will increase, and AI is very helpful in achieving this.
For example, the 0x0.ai project provides an artificial intelligence smart contract auditor, a tool that uses advanced algorithms to analyze smart contracts and identify potential vulnerabilities or problems that may lead to fraud or other security risks.Auditors use machine learning techniques to identify patterns and exceptions in the code, marking potential issues for further review.
In addition to the above three categories, there are also some native cases that use AI to help the Web3 field. For example, PAAL helps users create personalized AI bots, which can be deployed to Telegram and Discord to serve Web3 users; AI-driven multi-chain dex aggregationHera, which uses AI to provide the widest range of tokens and the best trading paths between any token pair. Overall, AI helps Web3 more as a tool-level helper.
Limitations and challenges of AI+Web3 projects
4.1 The actual obstacles in decentralized computing power
Among the current projects that help AI, a large part of the projects that support Web3 is focusing on decentralized computing power. It is a very interesting innovation to promote global users to become the computing power supply side through token incentives.On the one hand, there are also some practical problems that need to be solved:
Compared with centralized computing power service providers, decentralized computing power products usually rely on nodes and participants distributed around the world to provide computing resources.Because the network connections between these nodes may have latency and instability, the performance and stability may be worse than the centralized computing power product.
In addition, the availability of decentralized computing power products is affected by the degree of matching between supply and demand.If there are not enough suppliers or the demand is too high, it may lead to insufficient resources or inability to meet user needs.
Finally, decentralized computing power products usually involve more technical details and complexity than centralized computing power products.Users may need to understand and handle knowledge about distributed networks, smart contracts, and cryptocurrency payments, and the cost of user understanding and use will become higher.
After in-depth discussion with a large number of decentralized computing power projects, I found that the current decentralized computing power can only be limited to AI reasoning rather than AI training.
Next, I will use four small questions to help you understand the reasons behind it:
1. Why do most decentralized computing power projects choose to do AI reasoning instead of AI training?
2. Where is Nvidia awesome?What is the reason why decentralized computing power training is difficult?
3. What will the end of decentralized computing power (Render, Akash, io.net, etc.) look like?
4. What will the end of the decentralized algorithm (Bittensor) look like?
Next, let’s peel off the cocoon layer by layer:
1) Looking at this track, most decentralized computing power projects choose to do AI reasoning instead of training.The core is the different requirements for computing power and bandwidth.
To help everyone better understand, let’s compare AI to a student:
AI training: If we compare artificial intelligence to a student, then training is similar to providing artificial intelligence with a lot of knowledge, and examples can also be understood as data we often call it, and artificial intelligence learns from these knowledge examples.Since the nature of learning requires understanding and memory of a large amount of information, this process requires a lot of computing power and time.
AI reasoning: Then what is reasoning?It can be understood as using the knowledge learned to solve problems or take exams. In the reasoning stage, artificial intelligence uses the knowledge learned to solve them, rather than engaging in new knowledge, so the calculation amount required in the reasoning process is smaller..
It is easy to find that the difference in difficulty between the two is essentially that large model AI training requires a huge amount of data and the bandwidth required for high-speed data communication, so it is currently extremely difficult to implement decentralized computing power as training.However, the need for data and bandwidth inference is much smaller, and the possibility of implementation is greater.
For big models, the most important thing is stability. If training is interrupted, it needs to be retrained, and the cost of sunk is very high.On the other hand, the relatively low computing power requirement is possible, such as AI inference mentioned above, or training of vertical small and medium-sized models for some specific scenarios, which is possible in decentralization.There are some relatively large node service providers in the computing power network that can serve these relatively large computing power needs.
2) So where is the data and bandwidth jam point?Why is decentralized training difficult to achieve?
This involves two key elements of large model training: single card computing power and multiple cards are connected in parallel.
Single-card computing power: At present, all the centers that require training of big models, we call it supercomputing centers.To facilitate everyone’s understanding, we can use the human body as a metaphor. The supercomputer center is the human body’s tissue, and the underlying unit GPU is the cell.If the computing power of a single cell (GPU) is very strong, the overall computing power (single cell x number) may also be very strong.
Multi-card parallel connection: The training of a large model is often 100 billion GB. For supercomputer centers that train large models, at least one ten-thousand level A100 is required to be used as the base.Therefore, we need to mobilize these tens of thousands of cards for training. However, training of the big model is not simply connected, and it is not training on the second card after training on the first A100 card, but the difference of the modelIn part, training on different graphics cards may require results of B when training A, so multiple cards are involved in parallel.
Why is Nvidia so powerful and its market value has been taking off, but it is difficult for AMD and domestic Huawei and Horizon to catch up.The core is not the single-card computing power itself, but two aspects: CUDA software environment and NVLink multi-card communication.
On the one hand, it is very important whether there is a software ecosystem that can adapt to hardware, such as Nvidia’s CUDA system. Building a new system is difficult, just like building a new language, and the replacement cost is very high.
On the other hand, it is multi-card communication. In essence, the transmission between multiple cards is the input and output of information, how to connect it in parallel and how to transmit it.Because of the existence of NVLink, it is impossible to connect NVIDIA and AMD cards; in addition, NVLink will limit the physical distance between graphics cards and require graphics cards to be in the same supercomputing center, which leads to the decentralized computing power being distributed around the world.It’s more difficult to achieve.
The first point explains why AMD, Huawei and Horizon in China are currently difficult to catch up; the second point explains why decentralized training is difficult to achieve.
3) What will the end of decentralized computing power look like?
Decentralized computing power is currently difficult to train large models. The core is that the most important thing for large model training is stability. If training is interrupted, it needs to be retrained, and the cost of sunk is very high.Its requirements for parallel connection of multiple cards are very high, and the bandwidth is limited by physical distance.NVIDIA uses NVLink to realize multi-card communication. However, in a supercomputing center, NVLink will limit the physical distance between graphics cards, so the dispersed computing power cannot form a computing power cluster for large-scale training.
But on the other hand, the demand for relatively low computing power is possible, such as AI inference, or training of vertical small and medium-sized models in some specific scenarios, which is possible in decentralized computing power networks.When there are some relatively large node service providers, they have the potential to serve these relatively large computing power needs.As well as edge computing scenarios like rendering, it is also relatively easy to implement.
4) What will the end of the decentralized algorithm model look like?
The end of the decentralized algorithm model depends on the end of the future AI. I think the future AI battle may have 1-2 closed-source model giants (such as ChatGPT), plus a model with a blooming flower. In this context, There is no need to bind to one big model for the application layer product, but cooperates with multiple big models. In this context, Bittensor’s model has great potential.
4.2 The combination of AI+Web3 is relatively rough, and 1+1>2 is not achieved
Currently, among projects that combine Web3 with AI, especially in terms of AI to help Web3 projects, most projects still use AI on the surface and do not truly reflect the deep integration between AI and cryptocurrency.This kind of surface application is mainly reflected in the following two aspects:
-
First of all, whether it is using AI for data analysis and prediction, using AI in recommendation and search scenarios, or performing code audits, there is not much difference from the combination of Web2 projects and AI.These projects simply use AI to improve efficiency and analyze, without demonstrating native convergence and innovative solutions between AI and cryptocurrencies.
-
Secondly, many Web3 teams combine with AI more to purely utilize the concept of AI at the marketing level.They just used AI technology in very limited areas, and then began to promote the trend of AI, creating an illusion that projects are very close to AI.However, there are still a lot of gaps in these projects in terms of real innovation.
Although there are still these limitations in current Web3 and AI projects, we should realize that this is only an early stage of development.In the future, we can expect more in-depth research and innovation to achieve a closer integration between AI and cryptocurrency and create more native and meaningful solutions in areas such as finance, decentralized autonomous organizations, forecasting markets and NFTs.plan.
4.3 Token Economics becomes a buffer for AI project narrative
As the business model problem of AI projects mentioned at the beginning, as more and more large models have begun to gradually open source, a large number of AI+Web3 projects are often difficult to develop and raise funds in Web2, so we choose to superimpose the narrative of Web3.and token economics to facilitate user engagement.
But the real key is whether the integration of token economics can really help AI projects solve actual needs, or whether it is simply narrative or short-term value, it actually needs to be questioned.
At present, most AI+Web3 projects are far from reaching the practical stage. I hope that more down-to-earth and thoughtful teams can not only use tokens as a driving force for AI projects, but also truly meet the actual demand scenarios.
Summarize
At present, many cases and applications have emerged in AI+Web3 projects.First of all, AI technology can provide Web3 with more efficient and intelligent application scenarios.Through AI’s data analysis and prediction capabilities, Web3 users can have better tools in investment decision-making and other scenarios; in addition, AI can also audit smart contract code, optimize the execution process of smart contracts, improve the performance of blockchain andefficiency.At the same time, AI technology can also provide more accurate and intelligent recommendations and personalized services for decentralized applications to improve user experience.
At the same time, the decentralization and programmability of Web3 also provide new opportunities for the development of AI technology.Through token incentives, decentralized computing power projects provide new solutions to the dilemma of insufficient supply of AI computing power. Web3’s smart contracts and distributed storage mechanisms also provide a broader range of sharing and training of AI algorithms.Space and resources.Web3’s user autonomy and trust mechanisms have also brought new possibilities to the development of AI. Users can choose to participate in data sharing and training independently, thereby improving the diversity and quality of data and further improving the performance and accuracy of AI models.
Although the current AI+Web3 crossover project is still in its early stages and there are many difficulties to face, it also brings many advantages.For example, decentralized computing power products have some shortcomings, but they reduce their dependence on centralized institutions, provide greater transparency and auditability, and enable broader participation and innovation.Decentralized computing power products may be a valuable option for specific use cases and user needs; the same is true for data collection, and decentralized data collection projects also bring some advantages, such as reducing the dependence of a single data source., provide a wider data coverage and promote data diversity and inclusion, etc.In practice, these advantages and disadvantages need to be weighed and managed and technical measures are taken to overcome challenges to ensure that decentralized data collection projects have a positive impact on the development of AI.
In general, the integration of AI+Web3 provides unlimited possibilities for future technological innovation and economic development.By combining the intelligent analysis and decision-making capabilities of AI with the decentralization and user autonomy of Web3, we believe that in the future, we can build a smarter, more open and fairer economic and even social system.