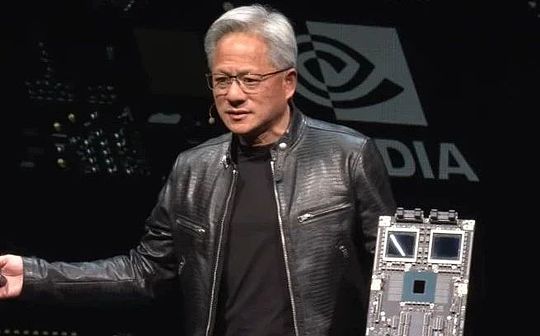
Source: Tencent Technology
Nvidia co-founder and CEO Huang Renxun delivered a keynote speech at Computex 2024 (2024 Taipei International Computer Exhibition), sharing how the era of artificial intelligence can promote the global new industrial revolution.
The following are the key points of this speech:
① Huang Renxun showed off the latest mass-produced version of Blackwell chips and said that he will launch Blackwell Ultra AI chips in 2025. The next generation of AI platform is named Rubin. Rubin Ultra will be launched in 2027. The update rhythm will be “once a year”, breaking “Moore”law”.
② Huang Renxun claimed that Nvidia promoted the birth of a large language model, which changed the GPU architecture after 2012 and integrated all new technologies on a single computer.
③ Nvidia’s accelerated computing technology has helped achieve a 100-fold increase in rate, while power consumption is only increased to 3 times, and the cost is 1.5 times.
④ Huang Renxun expects that the next generation of AI needs to understand the physical world.The method he gave is to let AI learn through video and synthetic data, and let AI learn from each other.
⑤ Huang Renxun even finalized a Chinese translation for token in PPT – Ci Yuan.
⑥ Huang Renxun said that the era of robots has arrived, and all moving objects will operate independently in the future.
The following is the full transcript of the two-hour speech compiled by Tencent Technology:
Dear guests, I am very honored to be here again.First of all, I would like to thank Taiwan University for providing us with this gymnasium as a venue for events.The last time I came here was when I got my degree from Taiwan University.Today, there is a lot of stuff we are going to explore, so I have to speed up and convey the message in a quick and clear way.We have a lot of topics to talk about, and I have a lot of exciting stories to share with you.
I am very happy to be here in Taiwan, China, where many of our partners are here.In fact, this is not only an indispensable part of NVIDIA’s development history, but also a key node for us and our partners to jointly promote innovation to the world.We work with many partners to build an artificial intelligence infrastructure worldwide.Today, I would like to discuss several key topics with you:
1) What progress is being made in our joint work and what is the significance of these progress?
2) What exactly is generative artificial intelligence?How will it affect our industry, and even every industry?
3) A blueprint on how we can move forward and how will we seize this incredible opportunity?
What will happen next?Generative AI and its profound impact, our strategic blueprint, are all exciting topics we are about to explore.We are standing at the starting point of the restart of the computer industry, and a new era created by you and created by you is about to begin.Now you are ready for the next important journey.
1.A new era of computing is beginning
But before starting the in-depth discussion, I want to emphasize one thing: Nvidia is located at the intersection of computer graphics, simulation and artificial intelligence, which forms the soul of our company.Today, everything I will show you is based on simulation.These are not just visual effects, they are the essence of mathematics, science and computer science, and the breathtaking computer architecture.No animation is pre-made, everything is a masterpiece of our own team.That’s what Nvidia understands, and we incorporate it all into the Omniverse virtual world we are proud of.Now, please enjoy the video!
Power consumption in data centers around the world is rising sharply, while computing costs are also rising.We are facing the severe challenge of computational inflation, which obviously cannot be maintained for a long time.Data will continue to grow exponentially, while CPU performance expansion is difficult to expand as quickly as before.However, there is a more efficient approach emerging.
For nearly two decades, we have been working on accelerated computing research.CUDA technology enhances the CPU’s capabilities, offloading and accelerating tasks that special processors can complete more efficiently.In fact, due to the slowdown or even stagnation of CPU performance expansion, the advantages of accelerated computing are becoming increasingly significant.I predict that every processing-intensive application will be accelerated, and in the near future, every data center will be fully accelerated.
Now, choosing accelerated computing is a wise move, which has become an industry consensus.Imagine that an application takes 100 units of time to complete.Whether it’s 100 seconds or 100 hours, we often can’t stand the AI application that runs for days or even months.
Among these 100 time units, one time unit involves code that needs to be executed sequentially. At this time, the importance of a single-threaded CPU is self-evident.The control logic of the operating system is indispensable and must be strictly executed in accordance with the instruction sequence.However, there are many algorithms, such as computer graphics, image processing, physical simulation, combinatorial optimization, graph processing and database processing, especially linear algebra widely used in deep learning, which are well suited for acceleration through parallel processing.To achieve this, we invented an innovative architecture that perfectly combines the GPU with the CPU.
A dedicated processor is able to speed up otherwise time-consuming tasks to incredible speeds.Since the two processors can work in parallel, they each run independently and independently.This means that tasks that originally required 100 units of time can now be completed in just 1 unit of time.Although this acceleration effect sounds incredible, today I will validate this statement with a series of examples.
The benefits of this performance improvement are amazing, with 100x acceleration, while only about 3x increase in power and only about 50% increase in cost.We have already practiced this strategy in the PC industry.Adding a $500 GeForce GPU to your PC will greatly improve its performance, while also increasing its overall value to $1,000.In the data center, we have adopted the same approach.A billion-dollar data center instantly transformed into a powerful artificial intelligence factory after adding a $500 million GPU.Today, this change is happening around the world.
The cost savings are equally shocking.For every $1 invested, you get up to 60 times the performance gain.Acceleration is 100 times, while power is only 3 times, and cost is only 1.5 times.The savings are real!
Apparently, many companies spend hundreds of millions of dollars on processing data in the cloud.Saving hundreds of millions of dollars becomes reasonable when data is processed faster.Why is this happening?The reason is simple, we have experienced a long-term efficiency bottleneck in general computing.
Now, we finally recognize this and decided to speed it up.By adopting a dedicated processor, we can regain a lot of previously overlooked performance gains, saving a lot of money and energy.That’s why I say, the more you buy, the more you save.
Now, I have shown you the numbers.Although they are not precise to a few decimal places, this accurately reflects the facts.This can be called “CEO Mathematics”.Although CEO mathematics does not pursue extreme precision, the logic behind it is correct – the more accelerated computing power you buy, the more cost you save.
2.350 function libraries help open up new markets
The results of accelerated computing are indeed extraordinary, but the implementation process is not easy.Why does it save so much money, but people haven’t adopted this technology earlier?The reason is that it is too difficult to implement.
There is no ready-made software that can be run simply by accelerating the compiler, and the application can be instantly speeded up by 100 times.This is neither logical nor realistic.If it were so easy, then CPU manufacturers would have done this long ago.
In fact, to achieve acceleration, the software must be fully rewrite.This is the most challenging part of the process.The software needs to be redesigned and recoded in order to convert algorithms that were originally running on the CPU into formats that can be run in parallel on the accelerator.
Although this computer science research is difficult, we have made significant progress over the past 20 years.For example, we launched the popular cuDNN deep learning library, which specializes in handling neural network acceleration.We also provide a library for artificial intelligence physics simulations for applications such as fluid dynamics that require compliance with physical laws.In addition, we have a new library called Aerial, which uses CUDA to accelerate 5G radio technology, allowing us to use software to define and accelerate telecommunications networks like software-defined Internet networks.
These acceleration capabilities not only improve performance, but also help us transform the entire telecom industry into a computing platform similar to cloud computing.In addition, the Coolitho computing lithography platform is also a good example, which greatly improves the efficiency of mask making, the most computationally intensive part of the chip manufacturing process.Companies such as TSMC have begun to use Coolitho for production, which not only significantly saves energy, but also significantly reduces costs.Their goal is to prepare for the further development of algorithms and the huge computing power required to make deeper and narrower transistors by accelerating the technology stack.
Pair of Bricks is our proud gene sequencing library, which has the world’s leading gene sequencing throughput.Co OPT is a remarkable combination optimization library that can solve complex problems such as route planning, itinerary optimization, and travel agency problems.It is generally believed that these problems need to be solved by quantum computers, but we have created an extremely fast algorithm through accelerated computing technology, successfully breaking 23 world records. To this day, we still maintain every major world record.
Coup Quantum is a quantum computer simulation system we developed.A reliable simulator is essential for researchers who want to design quantum computers or quantum algorithms.Without actual quantum computers, Nvidia CUDA – what we call the fastest computer in the world – became their preferred tool.We provide a simulator that can simulate the operation of quantum computers and help researchers make breakthroughs in the field of quantum computing.This simulator has been widely used by hundreds of thousands of researchers around the world and has been integrated into all leading quantum computing frameworks, providing strong support for scientific supercomputing centers around the world.
In addition, we have launched the data processing library Kudieff, which is specially designed to accelerate data processing.Data processing accounts for the vast majority of today’s cloud spending, so accelerating data processing is crucial to cost savings.QDF is an acceleration tool we developed that can significantly improve the performance of major data processing libraries in the world, such as Spark, Pandas, Polar and NetworkX graph processing databases.
These libraries are a key component of the ecosystem, and they enable accelerated computing to be widely used.Without our carefully crafted domain-specific libraries such as cuDNN, deep learning scientists around the world might not be able to fully utilize their potential with CUDA, because there are significant differences between CUDA and algorithms used in deep learning frameworks such as TensorFlow and PyTorch.This is as impractical as doing computer graphics without OpenGL or processing data without SQL.
These domain-specific libraries are treasures of our company and we currently have over 350 such libraries.It is these libraries that keep us open and ahead of the market.Today, I will show you more exciting examples.
Just last week, Google announced that they had deployed QDFs on the cloud and successfully accelerated Pandas.Pandas is the most popular data science library in the world, used by 10 million data scientists around the world, with monthly downloads of up to 170 million times.It’s like data scientists’ Excel, their right-hand assistant for processing data.
Now, just click on Google’s cloud data center platform Colab and you can experience the powerful performance brought by QDF-accelerated Pandas.This acceleration is really amazing, just like the demo you just saw, it completes the data processing task almost instantly.
3.CUDA realizes a virtuous cycle
CUDA has reached what people call a critical point, but the reality is better than that.CUDA has achieved a virtuous development cycle.Looking back at the history and the development of various computing architectures and platforms, we can find that such loops are not common.Take the microprocessor CPU as an example. It has been around for 60 years, but its accelerated computing has not fundamentally changed over the long years.
Creating a new computing platform often faces the dilemma of “there are chickens first or eggs first”.Without the support of developers, it is difficult for the platform to attract users; without the widespread adoption of users, it is difficult to form a huge installation foundation to attract developers.This dilemma has plagued the development of multiple computing platforms over the past 20 years.
However, we have successfully broken this dilemma by continuously rolling out domain-specific libraries and accelerated libraries.Today, we have 5 million developers around the world who use CUDA technology to serve almost every major industry and science field, from healthcare, financial services to the computer industry, the automotive industry.
As the customer base continues to expand, OEMs and cloud service providers have also begun to develop interest in our systems, which further drives more systems to enter the market.This virtuous cycle has created huge opportunities for us, allowing us to expand our scale and increase R&D investment, thereby promoting the accelerated development of more applications.
Acceleration of each application means a significant reduction in computing costs.As I’ve shown before, a 100x acceleration can bring up to 97.96%, or close to 98% cost savings.As we increase the calculation acceleration from 100 times to 200 times and then to 1000 times, the marginal cost of calculation continues to decline, showing remarkable economic benefits.
Of course, we believe that by significantly reducing computing costs, markets, developers, scientists and inventors will continue to unearth new algorithms that consume more computing resources.Until some point, a profound change will happen quietly.When the marginal cost of computing becomes so low, a brand new way of using computers will come into being.
In fact, this change is happening before our eyes.Over the past decade, we have used specific algorithms to reduce the marginal cost of computing by an astonishing 1 million times.Today, using all the data on the Internet to train large language models has become a logical and natural choice and is no longer questioned.
This idea – creating a computer that can process massive amounts of data and self-programming – is the cornerstone of the rise of artificial intelligence.The rise of artificial intelligence is possible entirely because we firmly believe that if we make computing cheaper, there will always be huge uses.Today, CUDA’s success has proved the feasibility of this virtuous cycle.
With the continuous expansion of the installation foundation and the continuous reduction of computing costs, more and more developers are able to realize their innovative potential and propose more ideas and solutions.This innovation has driven a surge in market demand.Now we are standing at a major turning point.However, before I show it further, I want to emphasize that what I want to show below would not be possible without the breakthrough of CUDA and modern AI technologies – especially generative AI.
This is the Earth 2 project—an ambitious idea to create the digital twin of the Earth.We will simulate the movement of the entire Earth to predict its future changes.Through such simulations, we can better prevent disasters and have a deeper understanding of the effects of climate change, allowing us to better adapt to these changes and even start changing our behaviors and habits now.
The Earth 2 project is probably one of the most challenging and ambitious projects in the world.We have made significant progress in this field every year, and this year’s results are particularly outstanding.Now, allow me to show you these exciting progress.
In the near future, we will have continuous weather forecasting capabilities covering every square kilometre on the planet.You will always understand how the climate will change, and this prediction will continue to run because we train AI, which requires extremely limited energy.It would be an incredible achievement.I hope you guys will enjoy it, and more importantly, this prediction was actually made by Jensen AI, not myself.I designed it, but the final predictions are presented by Jensen AI.
As we strive to continuously improve performance and reduce costs, researchers discovered CUDA in 2012, which was Nvidia’s first contact with artificial intelligence.That day is crucial for us because we made the wise choice to work closely with scientists to make deep learning possible.The emergence of AlexNet has achieved a huge breakthrough in computer vision.
4.The rise of AI supercomputers was not recognized at first
But the more important wisdom is that we take a step back and deeply understand the nature of deep learning.What is its basis?What is its long-term impact?What is its potential?We realize that this technology has great potential to continue to expand algorithms invented and discovered decades ago, combining more data, larger networks and crucial computing resources, deep learning can suddenly achieve humansTasks that algorithms cannot reach.
Now, imagine what would happen if we expanded our architecture further and had a larger network, more data and computing resources?So we are committed to reinventing everything.Since 2012, we have changed the architecture of the GPU, added the tensor core, invented NV-Link, launched cuDNN, TensorRT, Nickel, acquired Mellanox, and launched the Triton inference server.
These technologies are integrated on a brand new computer, which surpassed everyone’s imagination at the time.No one expected that no one made such a demand, and no one even understood its full potential.In fact, I’m not sure if anyone would want to buy it.
But at the GTC conference, we officially released this technology.A San Francisco startup called OpenAI quickly noticed our results and asked us to provide a device.I personally sent the world’s first artificial intelligence supercomputer DGX to OpenAI.
In 2016, we continued to expand our R&D scale.From a single artificial intelligence supercomputer to a single artificial intelligence application, it has expanded to the launch of a larger and powerful supercomputer in 2017.With the continuous advancement of technology, the world has witnessed the rise of Transformer.The emergence of this model allows us to process massive amounts of data and identify and learn continuous patterns over long spans.
Today, we have the ability to train these large language models to achieve major breakthroughs in natural language understanding.But we didn’t stop there, we continued to move forward and built a larger model.By November 2022, on extremely powerful artificial intelligence supercomputers, we use tens of thousands of Nvidia GPUs for training.
Just 5 days later, OpenAI announced that ChatGPT has 1 million users.This amazing growth rate has climbed to 100 million users in just two months, setting the fastest growth record in application history.The reason is very simple – ChatGPT’s user experience is convenient and magical.
Users can interact naturally and smoothly with computers, as if they are communicating with real people.Without tedious instructions or clear descriptions, ChatGPT can understand the user’s intentions and needs.
The emergence of ChatGPT marks an epoch-making change, and this slide captures this critical twist.Please allow me to show it for you.
It was not until the advent of ChatGPT that it truly revealed the infinite potential of generative artificial intelligence to the world.For a long time, the focus of artificial intelligence has focused on the fields of perception, such as natural language understanding, computer vision and speech recognition, technologies dedicated to simulating human perception abilities.But ChatGPT has brought a qualitative leap, not only limited to perception, but demonstrates the power of generative artificial intelligence for the first time.
It generates tokens one by one, which can be words, images, charts, tables, and even songs, text, voice and videos.A token can represent anything with clear meaning, whether it is chemicals, proteins, genes, or the weather patterns we mentioned earlier.
The rise of this generative artificial intelligence means that we can learn and simulate physical phenomena, allowing artificial intelligence models to understand and generate various phenomena in the physical world.We no longer limit ourselves to narrowing the scope and filtering, but instead explore infinite possibilities through generation.
Today, we can generate tokens for almost anything valuable, whether it is the steering wheel control of the car, the joint movement of the robotic arm, or anything we can learn at the moment.Therefore, we are no longer in an era of artificial intelligence, but a new era led by generative artificial intelligence.
More importantly, this device, which originally appeared as a supercomputer, has now evolved into an efficient and efficient artificial intelligence data center.It continues to produce, not only generates tokens, but also an artificial intelligence factory that creates value.This artificial intelligence factory is generating, creating and producing new commodities with huge market potential.
Just as Nikola Tesla invented the alternator at the end of the 19th century, bringing us a steady stream of electronics, Nvidia’s artificial intelligence generators are also continuously generating tokens with infinite possibilities.Both have huge market opportunities and are expected to change in every industry.This is indeed a new industrial revolution!
We are now welcoming a brand new factory that can produce unprecedented and valuable new products for all industries.This method is not only extremely scalable, but also completely repeatable.Please note that at present, various artificial intelligence models are constantly emerging every day, especially generative artificial intelligence models.Nowadays, every industry is competing to participate, which is an unprecedented grand occasion.
The $3 trillion IT industry is about to produce innovative achievements that can directly serve the $100 trillion industry.It is no longer just a tool for information storage or data processing, but an engine for generating intelligence in every industry.This will become a new type of manufacturing industry, but it is not a traditional computer manufacturing industry, but a new model of manufacturing using computers.Such a change has never happened before, and it is indeed a remarkable thing.
5.Generative AI promotes full-stack reshaping of software, demonstrating NIM cloud-native microservices
This opened a new era of accelerated computing, promoted the rapid development of artificial intelligence, and thus gave birth to the rise of generative artificial intelligence.And now, we are experiencing an industrial revolution.Let’s take a closer look at its impact.
The impact of this change is just as far-reaching for our industry.As I said before, this is the first time in the past sixty years, every layer of computing is undergoing transformation.From general-purpose computing of CPU to accelerated computing of GPUs, each change marks a leap in technology.
In the past, computers had to follow instructions to perform operations, but now, they deal more with LLM (big language model) and artificial intelligence models.Past computing models were based on retrieval, and almost every time you use your phone, it retrieves pre-stored text, images, or videos for you and recombines these contents according to the recommendation system to present them to you.
But in the future, your computer will generate as much content as possible and retrieve only the necessary information, because generating data consumes less energy when obtaining information.Moreover, the generated data has higher contextual relevance and can more accurately reflect your needs.When you need an answer, you no longer need to explicitly instruct the computer to “get me that information” or “give me that file”, just simply say, “give me an answer.”
Furthermore, computers are no longer just tools we use, they begin to generate skills.It performs tasks, and is no longer a production software industry, which was a disruptive idea in the early 1990s.Remember?The software packaging concept proposed by Microsoft has completely changed the PC industry.Without packaged software, our PC will lose most of its functionality.This innovation has driven the development of the entire industry.
Now we have a new factory, a new computer, and on this basis is a new type of software running – we call it Nim (NVIDIA Inference Microservices).Nim running in this new factory is a pre-trained model, which is an artificial intelligence.
This AI itself is quite complex, but the computing stack that runs AI is incredibly complex.When you use a model like ChatGPT, behind it is a huge software stack.This stack is complex and huge because the model has billions to trillions of parameters and runs not only on one computer, but works together on multiple computers.
To maximize efficiency, the system needs to allocate workloads to multiple GPUs for various parallel processing, such as tensor parallelism, pipeline parallelism, data parallelism, and expert parallelism.Such allocation is to ensure that work can be completed as quickly as possible, because in a plant, throughput is directly related to revenue, quality of service and the number of customers that can be served.Today, we are in an era where data center throughput utilization is crucial.
In the past, although throughput was considered important, it was not a decisive factor.However, now every parameter from startup time, run time, utilization, throughput to idle time is measured accurately because the data center has become a true “factory”.In this factory, operational efficiency is directly related to the company’s financial performance.
Given this complexity, we know the challenges most companies face when deploying AI.Therefore, we developed an integrated AI container solution that encapsulates AI in a box that is easy to deploy and manage.This box contains a huge collection of software such as CUDA, CUDACNN and TensorRT, as well as the Triton inference service.It supports cloud-native environments, allows automatic scaling in Kubernetes (a distributed architecture solution based on container technology) environment, and provides management services to facilitate users to monitor the operating status of artificial intelligence services.
What’s even more exciting is that this AI container provides a universal, standard API interface, allowing users to interact directly with the “box”.Users can easily deploy and manage AI services by simply downloading Nim and running on a CUDA-enabled computer.Today, CUDA is everywhere, it supports major cloud service providers, and almost all computer manufacturers provide CUDA support, and it can be found in hundreds of millions of PCs.
When you download Nim, you immediately have an AI assistant that communicates smoothly like a conversation with ChatGPT.Now, all the software has been streamlined and integrated into one container, and all the previously cumbersome 400 dependencies are centrally optimized.We conducted rigorous testing of Nim, and each pretrained model was fully tested on our cloud infrastructure, including different versions of GPUs such as Pascal, Ampere and the latest Hopper.These versions are of a wide variety, covering almost all requirements.
Nim’s invention is undoubtedly a feat, and it is one of my most proud achievements.Today, we have the ability to build large language models and various pre-trained models that cover multiple fields such as language, vision, imagery, and custom versions for specific industries such as healthcare and digital biology.
To learn more or try these versions, just visit ai.nvidia.com.Today, we released the fully optimized Llama 3 Nim on Hugging Face, which you can experience immediately and even take away for free.No matter which cloud platform you choose, you can run it easily.Of course, you can also download this container to your data center, host it yourself, and serve your customers.
As I mentioned earlier, we have Nim versions covering different fields, including physics, semantic search, visual language, etc., which support multiple languages.These microservices can be easily integrated into large applications, one of the most promising applications is the customer service agent.It is standard in almost every industry and represents a trillion-dollar global customer service market.
It is worth mentioning that nurses, as the core of customer service, play an important role in retail, fast food, financial services, insurance and other industries.Today, tens of millions of customer service staff have been significantly enhanced with the help of language models and artificial intelligence technology.At the heart of these enhancement tools is exactly what you see Nim.
Some are called Reasoning Agents, and when assigned tasks, they are able to identify goals and plan.Some are good at retrieving information, some are proficient in searching, and some may use tools such as Coop, or need to learn specific languages running on SAP such as ABAP, or even execute SQL queries.These so-called experts are now formed into an efficient and collaborative team.
The application layer has also changed: in the past, applications were written by instructions, but now, they are built by assembling artificial intelligence teams.While writing a program requires expertise, almost everyone knows how to break down problems and form a team.Therefore, I firmly believe that every company in the future will have a huge collection of Nim.You can select experts as you want and connect them into a team.
Even more amazing is that you don’t even need to figure out how to connect them.Just assign a task to the agent, Nim will intelligently decide how to break down the task and assign it to the best expert.They are like central leaders of applications or teams, able to coordinate the work of team members and ultimately present the results to you.
The whole process is as efficient and flexible as human teamwork.This is not just a trend in the future, but it is about to become a reality around us.This is a completely new look that applications will present in the future.
6.PC will become the main carrier of digital people
When we talk about interactions with large AI services, we can now do this with text and voice prompts.But looking forward to the future, we hope to interact in a more humane way, namely, digital people.Nvidia has made significant progress in the field of digital human technology.
Not only do digital people have the potential to be excellent interactive agents, they are more appealing and may show higher empathy.However, it still takes a huge effort to cross this incredible gap and make digital people look and feel more natural.This is not only our vision, but also our unremitting goal.
Before I show you our current achievements, please allow me to express my warm greetings to Taiwan, China.Before exploring the charm of night markets in depth, let us first appreciate the cutting-edge dynamics of digital human technology.
This is indeed incredible.ACE (Avatar Cloud Engine) not only runs efficiently in the cloud, but is also compatible with PC environments.We have forward-looking integration of Tensor Core GPUs into all RTX families, which marks the arrival of the era of AI GPUs and we are fully prepared for this.
The logic behind it is very clear: to build a new computing platform, a solid foundation must be laid first.With a solid foundation, applications will naturally emerge.If such a foundation is lacking, then the application will be out of the question.So, only when we build it can the prosperity of the application be possible.
Therefore, we have integrated Tensor Core processing units in every RTX GPU. Currently, 100 million GeForce RTX AI PCs have been put into use worldwide, and this number is still growing, and is expected to reach 200 million units.At the recent Computex exhibition, we launched four new AI laptops.
These devices have the ability to run artificial intelligence.Laptops and PCs of the future will become carriers of artificial intelligence, and they will silently provide you with help and support in the background.At the same time, these PCs will also run applications enhanced by artificial intelligence, and whether you are doing photo editing, writing, or using other tools, you will enjoy the convenience and enhancement effects brought by artificial intelligence.
In addition, your PC will be able to host digital human applications with artificial intelligence, allowing AI to be presented in more diverse ways and applied on your PC.Obviously, PC will become a crucial AI platform.So, how will we develop next?
I talked about the expansion of our data centers before, and each expansion is accompanied by new changes.When we expanded from DGX to large AI supercomputers, we implemented efficient training of Transformer on huge datasets.This marks a major shift: At the beginning, data requires human supervision, and artificial intelligence is trained through human markings.However, the amount of data that humans can tag is limited.Now, with the development of Transformer, unsupervised learning has become possible.
Today, Transformer is able to explore massive amounts of data, videos and images on its own, learning and discovering hidden patterns and relationships.In order to promote artificial intelligence to a higher level, the next generation of artificial intelligence needs to be rooted in the understanding of physical laws, but most artificial intelligence systems lack a deep understanding of the physical world.In order to generate realistic images, videos, 3D graphics, and simulate complex physical phenomena, we urgently need to develop physics-based artificial intelligence, which requires it to be able to understand and apply the laws of physics.
There are two main ways to achieve this.First, by learning from videos, artificial intelligence can gradually accumulate knowledge of the physical world.Secondly, using synthetic data, we can provide a rich and controllable learning environment for artificial intelligence systems.In addition, simulated learning between data and computers is also an effective strategy.This method is similar to AlphaGo’s self-game mode, allowing two entities with the same ability to learn from each other for a long time, thereby continuously improving their intelligence level.Therefore, we can foresee that this type of artificial intelligence will gradually emerge in the future.
7.Blackwell is fully put into production, and its computing power has increased by 1,000 times in eight years
When artificial intelligence data is generated through synthesis and combined with reinforcement learning technology, the rate of data generation will be significantly improved.As data generation grows, the demand for computing power will also increase accordingly.We are about to enter a new era in which artificial intelligence will be able to learn the laws of physics, understand and make decisions and actions based on data from the physical world.Therefore, we expect the AI model to continue to expand and the requirements for GPU performance will become increasingly high.
To meet this need, Blackwell came into being.Designed to support a new generation of artificial intelligence, this GPU has several key technologies.This chip size is the best in the industry.We use two chips as large as possible, connecting them tightly together through a high-speed link of 10 terabytes per second combined with the world’s most advanced SerDes (high-performance interface or connection technology).Further, we place two such chips on a computer node and coordinate efficiently through the Grace CPU.
Grace CPUs are versatile and not only suitable for training scenarios, but also play a key role in inference and generation, such as rapid checkpointing and restarting.In addition, it can store contexts, allowing AI systems to have memory and understand the context of user conversations, which is crucial to enhancing the continuity and fluency of interactions.
Our second-generation Transformer engine further improves the computing efficiency of artificial intelligence.This engine can dynamically adjust to lower accuracy according to the accuracy and range requirements of the computing layer, thereby reducing energy consumption while maintaining performance.Meanwhile, Blackwell GPUs also have secure artificial intelligence capabilities to ensure that users can ask service providers to protect them from theft or tampering.
In terms of GPU interconnection, we have adopted the fifth generation NV Link technology, which allows us to easily connect multiple GPUs.In addition, Blackwell GPUs are equipped with the first generation of reliability and availability engines (Ras systems), an innovative technology that can test every transistor, trigger, memory and off-chip memory on the chip to ensure that we can accurately judge on siteWhether a particular chip meets the average time between failures (MTBF).
Reliability is especially critical for large supercomputers.The average failure interval time for a supercomputer with 10,000 GPUs may be in hours, but when the number of GPUs increases to 100,000, the average failure interval time will be reduced to minutes.Therefore, to ensure that supercomputers can run stably for a long time to train complex models that may take months, we must improve reliability through technological innovation.The improvement of reliability can not only increase the uptime of the system, but also effectively reduce costs.
Finally, we also integrate an advanced decompression engine in the Blackwell GPU.In terms of data processing, decompression speed is crucial.By integrating this engine, we can pull data from storage 20 times faster than the existing technology, greatly improving data processing efficiency.
The above features of the Blackwell GPU make it a remarkable product.At the previous GTC conference, I showed you Blackwell in the prototype state.And now, we are pleased to announce that this product has been put into production.
This is Blackwell, everyone, using incredible technology.This is our masterpiece, the most complex and most performing computer in the world today.Among them, we particularly want to mention the Grace CPU, which carries huge computing power.Please see, these two Blackwell chips, they are closely connected.Have you noticed it?This is the largest chip in the world, and we use links up to A10TB per second to blend two such chips into one.
So, what exactly is Blackwell?Its performance is incredible.Please observe these data carefully.In just eight years, our computing power, floating-point arithmetic and artificial intelligence floating-point arithmetic capabilities have increased by 1,000 times.This speed almost surpasses Moore’s Law’s growth in the best period.
The growth in Blackwell’s computing power is simply amazing.What is more worth mentioning is that whenever our computing power increases, the cost is constantly declining.Let me show you something.By improving computing power, the energy used to train GPT-4 models (2 trillion parameters and 8 trillion tokens) has dropped by 350 times.
Imagine if Pascal does the same training, it would consume up to 1000 GWh of energy.This means that a GW data center is needed to support it, but such data centers do not exist in the world.Even if it exists, it will take a month to run continuously.And if it is a 100 MW data center, the training time will be as long as one year.
Obviously, no one wants or can create such a data center.That’s why eight years ago, big language models like ChatGPT were still a distant dream for us.But now, we achieve this by increasing performance and reducing energy consumption.
We used Blackwell to reduce the energy that would otherwise require up to 1,000 GWh to just 3 GWh, an achievement that is undoubtedly a shocking breakthrough.Imagine using 1,000 GPUs, the energy they consume is only equivalent to the calories of a cup of coffee.And 10,000 GPUs can complete the same task in just about 10 days.These progress made in eight years is simply incredible.
Blackwell is not only suitable for inference, but its improvement in token generation performance is even more eye-catching.In the Pascal era, each token consumed up to 17,000 joules of energy, which was about the energy of two light bulbs running for two days.To generate a GPT-4 token, it almost takes two 200-watt light bulbs to run for two days.Considering that it takes about 3 tokens to generate a word, this is indeed a huge energy consumption.
However, the situation is completely different now.Blackwell makes it only cost 0.4 joules of energy to generate each token, and token generation is at an amazing speed and extremely low energy consumption.This is undoubtedly a huge leap.But even so, we are still not satisfied.For a greater breakthrough, we must build more powerful machines.
This is our DGX system, and the Blackwell chip will be embedded in it.This system uses air cooling technology and is equipped with 8 such GPUs inside.Look at the heatsinks on these GPUs, their size is amazing.The power consumption of the entire system is about 15 kW, which is completely achieved through air cooling.This version is compatible with X86 and has been applied to our shipped servers.
However, if you prefer liquid cooling technology, we also have a brand new system – the MGX.It is based on this motherboard design, which we call a “modular” system.The core of the MGX system lies in two Blackwell chips, and each node integrates four Blackwell chips.It adopts liquid cooling technology to ensure efficient and stable operation.
In the entire system, there are nine such nodes, a total of 72 GPUs, forming a huge computing cluster.These GPUs are closely connected through the new NV link technology to form a seamless computing network.NV link switches are technical miracle.It is currently the most advanced switch in the world, with an astonishing data transmission rate.These switches make each Blackwell chip efficiently connected, forming a huge 72 GPU cluster.
What are the advantages of this cluster?First, in the GPU domain, it now acts like a single, super-large GPU.This “super GPU” has the core capabilities of 72 GPUs, and the performance is 9 times higher than the previous generation of 8 GPUs.At the same time, the bandwidth has increased by 18 times, the AI FLOPS (floating point operations per second) has increased by 45 times, and the power has increased by only 10 times.That is to say, one such system can provide strong power of 100 kilowatts, while the previous generation was only 10 kilowatts.
Of course, you can also connect more of these systems together to form a larger computing network.But the real miracle lies in the fact that this NV link chip is becoming increasingly large with the increasing size of the big language model.Because these large language models are no longer suitable for running on a single GPU or node, they require the working of the entire GPU rack together.Just like the new DGX system I just mentioned, it can accommodate large language models with hundreds of trillions of parameters.
The NV link switch itself is a technological miracle, with 50 billion transistors, 74 ports, and each port has a data rate of up to 400 GB.But more importantly, the switch also integrates mathematical operation functions, which can directly perform reduction operations, which is of great significance in deep learning.This is the new look of the current DGX system.
Many people expressed curiosity about us.They questioned that there was a misunderstanding of Nvidia’s business scope.People wonder how Nvidia could become so huge just by making GPUs.Therefore, many people have formed the impression that the GPU should look like in a certain way.
However, what I want to show you now is that this is indeed a GPU, but it is not the kind you think it is.This is one of the most advanced GPUs in the world, but it is mainly used in the gaming field.But we all know that the true power of GPUs is much more than that.
Everyone, please look at this, this is the true form of GPU.This is a DGX GPU designed for deep learning.The back of this GPU is connected to the NV link backbone, which consists of 5,000 lines and is 3 kilometers long.These lines are the NV link backbone, which connects 70 GPUs to form a powerful computing network.This is an electronic mechanical miracle in which the transceiver allows us to drive signals across the entire length on the copper wire.
Therefore, this NV link switch transmits data on the copper wire through the NV link backbone, allowing us to save 20 kW of power in a single rack, which is now fully used for data processing, which is indeed a fascinating factIncredible achievement.This is the power of the NV link backbone.
8.Promote Ethernet for Generative AI
But this is not enough to meet the demand, especially for large AI factories, so we have another solution.We have to connect these AI factories using high-speed networks.We have two network options: InfiniBand and Ethernet.Among them, InfiniBand has been widely used in supercomputing and artificial intelligence factories around the world and is growing rapidly.However, not every data center can use InfiniBand directly because they have made significant investments in the Ethernet ecosystem, and managing InfiniBand switches and networks does require some expertise and technology.
Therefore, our solution is to bring the performance of InfiniBand into the Ethernet architecture, which is not easy.The reason is that each node, each computer, is usually connected to different users on the Internet, but most of the communication actually occurs inside the data center, i.e. the data transmission between the data center and the users on the other end of the Internet.However, in the deep learning scenario of artificial intelligence factories, GPUs do not communicate with users on the Internet, but frequently and intensive data exchanges with each other.
They communicate with each other because they are all collecting part of the results.They then have to reduce these partial results and redistribute them.This communication mode is characterized by highly burst traffic.What matters is not the average throughput, but the last data that arrives, because if you are collecting partial results from everyone and I’m trying to receive all of your partial results, if the last packet arrives late, then the whole operation willDelay.Latency is a crucial issue for AI factories.
Therefore, our focus is not on average throughput, but on ensuring that the last packet arrives on time and without error.However, traditional Ethernet has not been optimized for such highly synchronized, low latency requirements.To meet this need, we creatively designed an end-to-end architecture that enables NICs (Network Interface Cards) and switches to communicate.To achieve this, we have adopted four key technologies:
First, Nvidia has the industry-leading RDMA (remote direct memory access) technology.Now we have RDMA at Ethernet network level and it does a great job.
Second, we introduced a congestion control mechanism.The switch has real-time telemetry function, which can quickly identify and respond to congestion in the network.When the amount of data sent by the GPU or NIC is too large, the switch immediately sends a signal to inform them to slow down the transmission rate, thereby effectively avoiding the generation of network hotspots.
Third, we adopt adaptive routing technology.Traditional Ethernet transmits data in a fixed order, but in our architecture we can flexibly adjust based on real-time network conditions.When congestion is found or some ports are idle, we can send packets to these idle ports and reorder them by the Bluefield device on the other end to ensure that the data is returned in the correct order.This adaptive routing technology greatly improves the flexibility and efficiency of the network.
Fourth, we implemented noise isolation technology.In a data center, noise and traffic generated by multiple models simultaneously training may interfere with each other and cause jitter.Our noise isolation technology can effectively isolate these noises, ensuring that the transmission of critical data packets is not affected.
By adopting these technologies, we have successfully provided high-performance, low-latency network solutions to AI factories.In a multi-billion-dollar data center, if network utilization increases by 40% and training time is reduced by 20%, this actually means that a $5 billion data center is equivalent to a $6 billion data center in performance, reveals the significant impact of network performance on overall cost-effectiveness.
Fortunately, Ethernet technology with Spectrum X is the key to our achievement, which greatly improves network performance, making network costs almost negligible relative to the entire data center.This is undoubtedly a great achievement we have made in the field of network technology.
We have a strong Ethernet product lineup, the most notable of which is the Spectrum X800.With 51.2 TB per second and 256 paths (radix) support, this device provides efficient network connectivity to thousands of GPUs.Next, we plan to launch the X800 Ultra in a year, which will support 512 radix with up to 512 paths, further improving network capacity and performance.The X1600 is designed for larger data centers and can meet the communication needs of millions of GPUs.
With the continuous advancement of technology, the data center era of millions of GPUs is just around the corner.There are profound reasons behind this trend.On the one hand, we are eager to train larger and more complex models; but more importantly, future Internet and computer interactions will increasingly rely on cloud-based generative artificial intelligence.These AIs will work and interact with us to generate videos, images, texts and even digital people.Therefore, almost every interaction we interact with computers is inseparable from the participation of generative artificial intelligence.And there is always a generative AI connected to it, some running locally, some running on your device, and many may run in the cloud.
These generative artificial intelligences not only have strong reasoning capabilities, but also iteratively optimize answers to improve the quality of answers.This means that we will generate massive data generation needs in the future.Tonight, we witnessed the power of this technological innovation together.
Blackwell, as the first generation of the NVIDIA platform, has attracted much attention since its launch.Today, the era of generative artificial intelligence is ushering in the world, the beginning of a new industrial revolution, and every corner is aware of the importance of artificial intelligence factories.We are deeply honored to have received extensive support from all walks of life, including every OEM (Original Equipment Manufacturer), computer manufacturer, CSP (Cloud Service Provider), GPU Cloud, sovereign cloud, and telecommunications companies.
Blackwell’s success, widespread adoption and industry enthusiasm for it have reached unprecedented levels, which is a great relief, and I would like to express my sincere gratitude to you.However, our pace will not stop.In this rapidly developing era, we will continue to work hard to improve product performance, reduce the cost of training and reasoning, while continuously expanding the capabilities of artificial intelligence so that every company can benefit from it.We firmly believe that with the improvement of performance, costs will be further reduced.The Hopper platform is undoubtedly the most successful data center processor in history.
9.Blackwell Ultra will be released next year, and the next generation platform is called Rubin
This is indeed a shocking success story.As you can see, the birth of the Blackwell platform is not a single component, but a complete system that integrates multiple elements such as CPU, GPU, NVLink, NICK (specific technical components) and NVLink switches.We are committed to tightly connecting all GPUs through each generation using large, ultra-high-speed switches to form a huge and efficient computing domain.
We integrate the entire platform into our AI factory, but more critically, we provide this platform to customers around the world in a modular form.The original intention of this is that we expect every partner to create a unique and innovative configuration based on their own needs to adapt to different styles of data centers, different customer groups and diverse application scenarios.From edge computing to telecommunications, all kinds of innovations will become possible as long as the system remains open.
To enable you to innovate freely, we have designed an integrated platform, but at the same time provided to you in decomposition, allowing you to easily build modular systems.Now, the Blackwell platform has been fully launched.
Nvidia always adheres to the annual update rhythm.Our core philosophy is very clear: 1) build solutions that cover the entire data center scale; 2) break these solutions into components and launch them to customers around the world at an annual frequency; 3) We spare no effort to push all technologies towardExtreme, whether it is TSMC’s process technology, packaging technology, memory technology, or optical technology, we all pursue ultimate performance.
After completing the ultimate challenge of hardware, we will do our best to ensure that all software runs smoothly on this complete platform.In computer technology, software inertia is crucial.When our computer platform is backward compatible and the architecture is perfectly compatible with existing software, the speed of the product will be significantly improved.Therefore, when the Blackwell platform was launched, we were able to make full use of the built software ecosystem foundation to achieve amazing market response speed.Next year, we will welcome the Blackwell Ultra.
Just like the H100 and H200 series we have launched, the Blackwell Ultra will also lead the craze of a new generation of products, bringing unprecedented innovative experiences.At the same time, we will continue to challenge the limits of technology and launch the next-generation spectrum switch, which is the first attempt in the industry.This major breakthrough has been successfully achieved, although I am still a little hesitant to make this decision public.
Within Nvidia, we are used to using code names and maintaining some confidentiality.Many times, even most employees in the company do not know these secrets very well.However, our next-generation platform has been named Rubin.I won’t go into details about Rubin here.I know everyone’s curiosity, but please allow me to keep some mystery.You may be eager to take photos or study those small characters carefully, so feel free to do so.
We not only have the Rubin platform, but we will also launch the Rubin Ultra platform in a year.All the chips shown here are in full development stage, ensuring that every detail is carefully polished.Our update pace is still once a year, always pursuing the ultimate in technology while ensuring that all products maintain 100% architectural compatibility.
Looking back on the past 12 years, from the moment Imagenet was born, we foresee the future of the computing field that will undergo earth-shaking changes.Now, all this has become a reality, which coincides with our original idea.From GeForce before 2012 to Nvidia today, the company has undergone a huge transformation.Here, I would like to sincerely thank all my partners for their support and company along the way.
10.The era of robots has arrived
This is Nvidia’s Blackwell platform. Next, let’s talk about the future of combining artificial intelligence with robots.
Physical artificial intelligence is leading a new wave in the field of artificial intelligence. They are well versed in the laws of physics and can easily integrate into our daily lives.To this end, physical artificial intelligence not only needs to build an accurate world model to understand how to interpret and perceive the world around it, but also needs to have excellent cognitive abilities to deeply understand our needs and perform tasks efficiently.
Looking ahead, robotics will no longer be an out of reach concept, but will be increasingly integrated into our daily lives.When talking about robotics, people often think of humanoid robots, but in fact, their applications are much more than that.Mechanization will become the norm, factories will fully realize automation, and robots will work together to create a series of mechanized products.The interaction between them will be closer, creating a highly automated production environment together.
To achieve this, we need to overcome a range of technical challenges.Next, I will showcase these cutting-edge technologies through video.
This is not just a vision for the future, it is gradually becoming a reality.
We will serve the market in a variety of ways.First, we are committed to creating platforms for different types of robot systems: dedicated platforms for robot factories and warehouses, object manipulation robot platforms, mobile robot platforms, and humanoid robot platforms.Like many of our other businesses, these robotic platforms rely on computer acceleration libraries and pre-trained models.
We use computer acceleration libraries, pre-trained models, and conduct comprehensive testing, training and integration in Omniverse.As the video shows, Omniverse is where robots learn how to better adapt to the real world.Of course, the ecosystem of robot warehouses is extremely complex and requires many companies, tools and technologies to jointly build modern warehouses.Today, warehouses are gradually moving towards full mechanization and will one day be fully automated.
In such an ecosystem, we provide SDK and API interfaces for the software industry, edge artificial intelligence industry and companies, and also design dedicated systems for PLC and robotic systems to meet the needs of specific fields such as the Ministry of Defense.These systems are integrated through integrators to ultimately create efficient and intelligent warehouses for customers.For example, Ken Mac is building a robot warehouse for the Giant Giant Group.
Next, let’s focus on the factory field.The ecosystem of the factory is very different.Take Foxconn as an example, they are building some of the world’s most advanced factories.The ecosystems of these factories also cover edge computers, robotic software, for designing factory layouts, optimizing workflows, programming robots, and PLC computers used to coordinate digital factories and artificial intelligence factories.We also provide an SDK interface for every link in these ecosystems.
Such changes are taking place worldwide.Foxconn and Delta are building digital twins for their factories to achieve a perfect blend of reality and digital, and Omniverse plays a crucial role.It is also worth mentioning that Pegatron and Wistron are also following the trend and establishing digital twin facilities for their respective robot factories.
This is really exciting.Next, please enjoy a wonderful video of Foxconn’s new factory.
The robot factory consists of three main computer systems that train AI models on the NVIDIA AI platform, and we ensure that the robot runs efficiently on the local system to orchestrate the factory processes.At the same time, we use Omniverse, a simulation collaboration platform to simulate all factory elements including robotic arms and AMR (autonomous mobile robot).It is worth mentioning that these simulation systems share the same virtual space to achieve seamless interaction and collaboration.
When the robotic arms and AMR enter this shared virtual space, they can simulate a real factory environment in Omniverse, ensuring adequate verification and optimization before actual deployment.
To further enhance the integration and application range of solutions, we offer three high-performance computers, equipped with acceleration layers and pre-trained AI models.In addition, we have successfully combined NVIDIA Manipulator and Omniverse with Siemens’ industrial automation software and systems.This collaboration allows Siemens to enable more efficient robot operation and automation in factories around the world.
In addition to Siemens, we have also established cooperative relationships with many well-known companies.For example, Symantec Pick AI has integrated NVIDIA Isaac Manipulator, while Somatic Pick AI has successfully run and operated robots from well-known brands such as ABB, KUKA, and Yaskawa Motoman.
The era of robotics and physical artificial intelligence has arrived, and they are being widely used everywhere. This is not science fiction, but reality, which is exciting.Looking ahead, robots in factories will become mainstream, and they will make all the products, two of which are particularly eye-catching.First, autonomous cars or cars with high autonomy, Nvidia once again plays a central role in this field with its comprehensive technology stack.Next year, we plan to join hands with the Mercedes-Benz team and then work with the Jaguar Land Rover (JLR) team in 2026.We offer a complete solution stack, but customers can choose any part or hierarchy based on their needs, as the entire driver stack is open and flexible.
Next, another product that may be manufactured at high yields from robot factories is humanoid robots.In recent years, great breakthroughs have been made in cognitive ability and world understanding ability, and the development prospects in this field are exciting.I’m particularly excited about humanoid robots because they are most likely to adapt to the world we have built for humans.
Training humanoid robots requires a lot of data compared to other types of robots.Since we have similar body shapes, the large amount of training data provided through demonstration and video capabilities will be of great value.Therefore, we expect significant progress in this area.
Now, let’s welcome some special robot friends.The era of robots has arrived, and this is the next wave of artificial intelligence.There are a wide variety of computers made in Taiwan, including traditional models equipped with keyboards, small, light and portable mobile devices, as well as professional equipment that provides powerful computing power for cloud data centers.But looking forward, we will witness a more exciting moment – creating computers that can walk and roll around, namely intelligent robots.
These intelligent robots have striking technical similarities to computers as we know them, and they are all built on advanced hardware and software technologies.So, we have reason to believe that this will be a truly extraordinary journey!