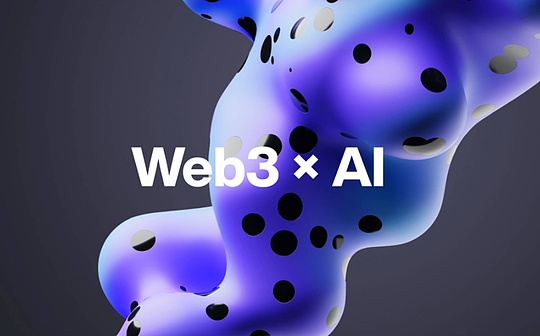
Author: Haotian Source: X,@HAOTIANCRYPTOINSIGHT
Everyone is hoping that AI+Web3 will become the catalyst for this bull market, which can be seen from the high valuation and heavy bets given by VC.The question is, what are the problems currently in the AI+Web3 fusion track?Combined with this systematic report by @web3caff_zh, let me talk about my views:
1) AI training requires large-scale data, and Web3 is used to do data tracking and the incentive effects derived from it.In the long run, AI will inevitably need the help of web3, but it is necessary to clarify that web3 can only solve the limited problems of AI.
For example, the main driving force in core fields such as traditional large-scale data training, continuous algorithm optimization, computer vision, speech recognition technology, game AI, etc., also depends on large-scale concentrated computing power and hardware adaptation optimization such as chips and algorithms, such as depth.Learning convolutional neural networks, reinforcement learning, brain-like computing models and other directions to expand the boundaries of AI capabilities will not be possible for web3 to gain a foothold in the short term;
2) Generative AI only accounts for a small branch of the AI major sector, but it accelerates the integration of AI and web3.Because generative AI is an AI universal technology that is more application-oriented.Ideally, the basic big model will generally be handled by large companies using concentrated computing power and adopt open source policies to drive their upper-level application market.The overall AI market will gradually become longer-tailed, and the importance of model fine-tuning and reasoning will be highlighted.
However, once a company that has mastered core computing power and model resources changes its open source policy, it will have a direct impact on the overall AI market. To avoid this crisis, an infra that relies more on distributed computing power architecture and distributed inference collaboration architecture willBecome a must.
3) web3 can play a key role in the construction of AI distributed frameworks. For example: during model training, blockchain can create unique identifiers for data sources, and do data deduplication to improve training efficiency; when computing power is insufficient,Blockchain can use the Tokenomics incentive mechanism to build a distributed AI computing power network; in the parameter fine-tuning process, blockchain can record different versions of the model, track the evolution of the model and perform fine-grained control;
In the model inference process, ZK, TEE and other technologies can be used to build a decentralized inference network to enhance communication and mutual trust between models; in the edge computing and DePIN integration process, web3 can help build a decentralized edge AI network and drive AI+The combination of DePIN Internet of Things.
4) When talking about the combination point of AI+Web3, Vitalik stated that AI can be integrated step by step as a participant in the Web3 world, so the integration of AI and web3 will definitely be very slow.
On the one hand, the mainstream web2 world’s attention is still at the level of AI display performance, but does not rely much on the AI behind-the-scenes collaboration framework, and there is a problem of being disconnected from web3; on the other hand, web3 is still in distributed computing in the field of AI integration.The construction stage of basic infra, such as the power network, distributed inference architecture network, distributed Tokenomics application network, distributed AI Agent tool collaboration network, etc., has not been fully verified and applied by the mainstream web2 urgent needs group.
In short, in a word, the general trend of AI+Web3 is correct, but the actual implementation is not so fast. It may take one cycle or even cross cycles to see significant progress, and it requires a little more patience.