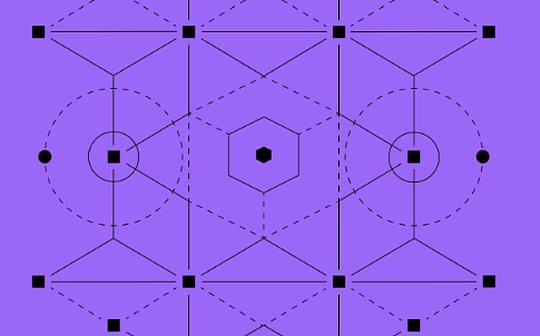
Author: Lucas TCHEYAN, Associate Researcher Galaxy; Translation: 0xjs@作 作 作 作 作 作
Foreword
The emergence of the public chain is one of the deepest progress in the history of computer science.But the development of AI will have a profound impact on our world.If blockchain technology provides new templates for transaction settlement, data storage and system design, then artificial intelligence is a revolution in computing, analysis and content delivery.The innovation of these two industries is release new use cases, which may accelerate the use of these two industries in the next few years.This report explores the continuous integration of cryptocurrencies and AI, and focuses on the novel cases that try to bridge the gap between the two and use the strength of the two.Specifically,This report has studied the development decentralized calculation agreement, zero -knowledge machine learning (ZKML) infrastructure and AI intelligence projects.
Cryptocurrencies provide AI with a settlement layer without permission, no trust, and combined.This unlocks the use case, such as the decentralized computing system to make the hardware easier to access. Construct a complex AI intelligent body that can execute value exchanges, as well as the development identity and the Provenance solution to fight against witch attacks and in -depth falsification.AI brings many of the same benefits we see in Web 2 to cryptocurrencies.This includes a user experience (UX) and potential potential for users and developers that have enhanced the user and developers due to the specially trained ChatGPT and Copilot versions) for users and developers.Blockchain is a transparent data rich environment required by AI.However, the computing power of the blockchain is also limited, which is the main obstacle to the AI model directly.
Experiments in the fields of cryptocurrencies and AI cross -fields and the driving force behind the final use of the most promising use cases to promote cryptocurrencies are the same -access to coordination layers that do not require licenses and no trust to better promote the transfer of value.In view of the huge potential, participants in this field need to understand the basic ways of crossing these two technologies.
Key points:
-
In the near future (6 months to 1 year), the integration of cryptocurrencies and AI will be led by AI applicationsThese applications can improve the efficiency of developers, auditability and security of smart contracts, and user accessibility.These integrations are not specific to cryptocurrencies, but to enhance the developers and user experience on the chain.
-
Just as a high -performance GPU serious shortage,Decentralization computing products are implementing AI custom GPU productsIt provides a driving force for adoption.
-
User experience and supervision are still the obstacles to attract decentralization to calculate customers.However, OPENAI’s latest development and the ongoing regulatory review of the United States highlight the value proposition of the AI network that is not required, anti -censorship, and decentralization.
-
AI integrated on the chain, especially smart contracts that can use artificial intelligence models, need to improve the calculation method calculated by ZKML technology and other verification chains.The lack of comprehensive tools and developers and high costs are obstacles to adopt.
-
AI Smart is very suitable for cryptocurrenciesUsers (or intelligence itself) can create wallets to trade with other services, intelligence or personnel.Currently using traditional financial methods cannot be achieved.In order to be more widely used, it is necessary to integrate additional integration with non -encrypted products.
the term
AIIt is the ability to use calculations and machines to imitate human reasoning and solve problems.
Neural networkIt is a training method for artificial intelligence models.They run input through the discrete algorithm layer and improve them until the required output is generated.The neural network consists of a heavy equation and can modify the weight to change the output.They may require a lot of data and calculations for training so that their output is accurate.This is one of the most common ways to develop the AI model (ChatGPT uses the process of neural network depends on the Transformer’s neural network).
trainIt is the process of developing neural networks and other artificial intelligence models.It requires a lot of data to train the model to correctly explain the input and generate accurate output.During the training process, the weight of the model equation is constantly modified until a satisfactory output is generated.Training costs may be very expensive.For example, ChatGPT uses tens of thousands of own GPUs to process data.Teams with less resources usually depend on specialized computing providers, such as Amazon Web Services, Azure and Google Cloud.
reasoningIt is the actual use of the AI model to obtain the output or result (for example, using ChatGPT to create an outline of the paper on the crossover of cryptocurrencies and AI).Reasoning will be used in the entire training process and final products.Due to the cost of calculating costs, even after the training is completed, their operating costs may be very high, but their calculation intensity is lower than training.
Zero Knowledge Proof (ZKP)Make a verification declaration without leaking basic information.This is very useful in cryptocurrencies, mainly two reasons: 1) privacy and 2) expansion.In order to protect privacy, it can make transactions without leaking sensitive information (such as how many ETHs in Wallets).For expansion, it enables the new execution calculation of the chain to be proven faster on the chain.This enables blockchain and applications to calculate cheaply under the chain, and then verify them on the chain.
AI/cryptocurrency ecological map
AI and cryptocurrencies are still constructing underlying infrastructure that supports large -scale chains on large -scale chains.
Decentralization computing marketIt is rising to provide a large number of physical hardware required for training and reasoning artificial intelligence models, mainly in the form of GPU.These two -way markets connect those who lease and seek rental calculations to promote the transfer and computing of value.In the decentralization calculation, several sub -categories that are providing additional functions are appearing.In addition to bilateral markets, this report will also review machine learning training providers that can provide verified training and fine -tuning output, and projects committed to connecting computing and model generation to implement AI. They are also often called intelligent incentives.
zkmlIt is hoped to provide an emerging key areas of projects that can provide verified model output in an effective and timely way of economy.These projects mainly enable applications to process heavy computing requests under the chain, and then publish verified outputs on the chain to prove that the workload under the chain is complete and accurate.ZKML is expensive and time -consuming in the current instance, but more and more is used as a solution.This is obvious in the increasing number of integration between the ZKML provider and the DEFI/game application who wants to use the AI model.
The ability of sufficient calculation and verification chain isAI Smart on the ChainOpen the doorEssenceSmart body is a trained model that can represent users’ execution requests.The smart body provides a significant opportunity to enhance the chain experience. Users can perform complex transactions with a conversation with chat robots.However, as far as the current is concerned, smart projects are still focusing on the development of infrastructure and tools to achieve easy and fast deployment.
Decentralization calculation
Overview
AI requires a lot of calculations to train models and operate reasoning.In the past ten years, as the model has become more and more complicated, the calculation needs have grown index.For example, OPENAI found that from 2012 to 2018, the computing demand of its model changed from two years to every three and a half months.This has led to a surge in demand for GPUs, and some cryptocurrency miners even re -use their GPUs to provide cloud computing services.With the intensification and increased cost of access computing, some projects are using encryption technology to provide decentralized computing solutions.They are calculated on demand at a competitive price so that the team can train and run the model in an affordable way.In some cases, weighing performance and security.
The most advanced GPU (such as GPU produced by NVIDIA) is very demanding.In September 2023, Tether acquired Northern Data, Germany’s Bitcoin miner Northern Data. According to reports, the company spent $ 420 million to purchase 10,000 H100 GPUs (one of the most advanced GPUs for AI training).The waiting time for first -class hardware may be at least six months, and even longer in many cases.To make matters worse, the company is often required to sign long -term contracts to obtain the calculation that they may not even use.This may lead to the existence of available calculations but unavailable in the market.The decentralized computing system helps solve the problem of low efficiency of these markets. Create a secondary market. The calculation owner can immediately transfer its excess capacity after receiving the notice, thereby releasing new supply.
In addition to competitive pricing and accessibility, the key value proposition of decentralized calculation is anti -review.Top AI development is increasingly led by large technology companies with unparalleled computing and data access capabilities.The first key theme emphasized in the AI Index Report in 2023 is that the industrial industry has increasingly surpassed the academic circles in the development of AI models, and concentrated control in the hands of a few technical leaders.This has aroused concerns about whether they have the ability to have a huge influence in formulating the specifications and values of supporting AI models, especially after these technology companies promote supervision to restrict their unprepared artificial intelligence development.
Decentralization calculation vertical field
In recent years, several decentralized computing models have appeared, and each model has its own focus and balance.
General computing
Akash, IO.NET, IEXEC, CUDOSThe projects are decentralized computing applications. In addition to data and general -purpose computing solutions, they also provide or are about to provide access permissions for special calculations for AI training and reasoning.
Akash is currently the only complete “super cloud” platform.It is a proof of equity of the COSMOS SDK.AKT is the native currency of AKASH, as a form of payment to protect network security and inspire participation.Akash launched the first main network in 2020, focusing on providing a cloud computing market without permission. It was originally characterized by storage and CPU leasing services.In June 2023, Akash launched a new test network focusing on GPU, and launched the GPU main network in September. Users can rent GPU for artificial intelligence training and reasoning.
There are two main participants in the Akash ecosystem -tenants and suppliers.Practitioners are users who want to buy AKASH network computing resources.Suppliers are computing resource suppliers.In order to match tenants and suppliers, Akash relies on the reverse auction process.Tenants submit their computing requirements, in which they can specify certain conditions, such as the position of the server or the hardware type calculated, and the amount they are willing to pay.Then, the supplier submits their asking price, and the minimum bid will get the task.
Akash verifications maintain the integrity of the network.The authentication set is currently limited to 100, and plans to gradually increase over time.Anyone can become verified as more AKTs by pledged more AKTs by pledged more AKT.AKT holders can also entrust their AKT to verifications.The trading costs and block rewards of the network are allocated in the form of AKT.In addition, for each lease, the AKASH network will earn “charging fees” at a rate determined by the community and distribute it to AKT holders.
Secondary market
The decentralized computing market aims to fill the existing computing market’s low efficiency.The supply limit causes the company to hoard the computing resources that may be exceeded, and the customer locks the customer in the long -term contract due to the contract structure with the cloud provider.The decentralized computing platform releases a new supply, so that anyone in the world with computing needs can become suppliers.
The surge in the surge in the GPU demand for AI training will be transformed into long -term network use on AKash.For example, AKASH has been providing a market for the CPU for a long time, providing similar services to centralized alternatives with 70-80% discounts.However, the lower price does not bring significant use.The active leases on the Internet have become gentle. By the second quarter of 2023, the average calculation of only 33%, 16% of memory and 13% storage.Although these are impressive indicators used on the chain (as a reference, the leading storage provider Filecoin has storage utilization rate of 12.6%in the third quarter of 2023), which indicates that the supply of these products continues to exceed demand.
Akash has launched the GPU network for more than half a year, and it is too early to accurately evaluate the long -term adoption rate.So far, the average utilization rate of the GPU is 44%, which is higher than the CPU, memory and storage, which is a sign of demand.This is mainly driven by the demand for the highest quality GPU (such as A100), and more than 90%has been rented.
Akash’s daily expenditure has also increased, which has almost doubled compared to the GPU.This part is due to the increase in other service usage, especially the CPU, but it is mainly the result of the new GPU usage.
The pricing is equivalent to centralized competitors such as Lambda Cloud and Vast.ai (or even more expensive in some cases).The huge demand for the highest -end GPU (such as H100 and A100) means that most of the owners of the device are not interested in listing in a market for competitive pricing.
Although the initial interest is very promising, there are still obstacles (further discussion below).The decentralized computing network requires more measures to generate demand and supply, and the team is trying to attract new users.For example, in early 2024, Akash passed the No. 240 proposal to increase the AKT emissions of the GPU supplier and inspire more supply, especially for high -end GPUs.The team is also committed to launching a concept verification model to show the real -time function of its network to potential users.Akash is training their own basic models, and has launched chat robots and image generation products, which can use Akash GPU to create output.Similarly, IO.NET has developed the Stable Diffusion model and is launching new network functions to better imitate the performance and scale of traditional GPU data centers.
Decentralized machine learning training
In addition to the universal computing platform that can meet the needs of AI, a group of professional AI GPU suppliers focusing on machine learning model training are also emerging.For example,GenesynThe point of view is “coordinating electricity and hardware to build collective wisdom.” The point of view is that “if someone wants to train something and someone is willing to train it, this training should be allowed.”
There are four main participants in the protocol: submitters (Submitters), Solvers, Verifiers, and WhistleBlowersEssenceSubmitter submits the task of training requests with training requests to the Internet.These tasks include training goals, models and training data to be trained.As part of the submission process, the submitters need to pay the pre -payment fee for the estimated amount required for the solution.
After submitting, the task will be assigned to the solution to practical training for the model.The solution then submits the completed tasks to the verification person, and the verification person is responsible for checking the training to ensure the correct completion.The whistle is responsible for ensuring that the verified person is honest.In order to motivate whistlers to participate in the network, Gensyn plans to regularly provide deliberate error evidence to reward whistlers to catch them.
In addition to providing computing for artificial intelligence -related workloads, the key value proposition of Gensynyn is its verification system, which is still under development.To ensure the correct execution of the external computing of the GPU supplier (that is, to ensure that the user’s model is trained in the way they want), verification is necessary.Gensyn uses a unique method to solve this problem, using a novel verification method called “probability learning certificate, graphic accurate protocols, and TrueBit incentive games”.This is a optimistic solution mode that allows verifications to confirm that the solution has correctly run the model without having to completely re -run the model by themselves. It is a high -cost and inefficient process.
In addition to its innovative verification method, Gensyn also claims that it is cost -effective compared to centralized alternatives and cryptocurrency competitors. The ML training price provided is as high as 80%cheaper than AWS.project.
Whether these preliminary results can be replicated in a decentralized network remains to be observed.Gensyn hopes to use the excess computing power of providers such as small data centers, retail users, and future mobile devices such as small mobile devices.However, as the Gensyn team itself recognizes, dependent heterogeneous computing providers have brought some new challenges.
For centralized suppliers such as Google Cloud Provers and Coreweave, the cost is expensive, and the communication (bandwidth and delay) between calculations is cheap.These systems aim to achieve communication between hardware as soon as possible.Gensyn has subverted this framework and allows anyone in the world to provide GPU to reduce calculation costs, but at the same time, it also increases communication costs, because the network must now coordinate and calculate operations on the heterogeneous hardware that is far apart.Gensyn has not been launched, but it is a conceptual proof that may be implemented when building a decentralized machine learning training agreement.
Decentralized universal intelligence
The decentralized computing platform also provides the possibility for the design of the AI creation method.BittersorIt is a decentralized calculation agreement based on Substrate, trying to answer “how do we turn AI into a collaborative method?”Bittensor aims to achieve decentralization and commercialization generated by AI.The agreement was launched in 2021, hoping to use the power of the collaborative machine learning model to continuously iterate and produce better AI.
Bittersor draws inspiration from Bitcoin. The supply of native currency TAO is 21 million, a half -cycle of four years (the first halved will be in 2025).Bittersor does not use the workload proof to generate the correct random number and obtain block rewards, but depends on the “Proof of Intelligence”, which requires miners to run models to respond to reasoning requests and generate output.
Inspiring intelligence
Bittensor originally relied on expert mixed (MOE) model to generate output.When submitting a reasoning request, the MOE model will not rely on a broad model, but to forward the reasoning request to the most accurate model of the given input type.Imagine the construction of a house, and you hire a variety of experts to be responsible for the differences in the construction process (for example: architects, engineers, paint workers, construction workers, etc.).MOE applies it to a machine learning model and tries to use the output of different models according to the input.As the founder of Bittensor explained by Ala Shaabana, this is like “talking to a smart person in a house and getting the best answer, not talking to a person.”Because there are challenges in ensuring the correct route, the synchronization of the message to the correct model, and the incentives, this method has been put on hold until the project is further developed.
There are two main participants in the Bittensor network: verifications and miners.The task of the verifications is to send reasoning requests to the miners, review their output, and rank them according to their response quality.In order to ensure that their rankings are reliable, the verifications will give the “VTRUST” score based on their ranking and other verification rankings.The higher the VTRUST score of the verification, the more TAO they get.This is to encourage the verifications to reach a consensus on the model ranking over time, because the more verified by the ranking, the higher their personal VTRUST score.
Miners, also known as the service, are network participants who run the actual machine learning model.Miners compete with each other to provide verifiers with the most accurate output for given queries. The more accurate the output, the more Tao earned.Miners can generate these outputs as they want.For example, in the future, Bittersor miners are completely likely to train models on Gensyn before and use them to earn Tao.
Nowadays, most interactions occur directly between verificationrs and miners.The verification person submits the input to the miner and requests the output (that is, the training model).Once the verificationrs query the miners on the network and receive their response, they will rank miners and submit them to the network.
This interaction between verification (dependent POS) and miners (dependent model proves, a form of POW) is called Yuma consensus.It aims to motivate miners to produce the best output and earn TAO, and motivate verifications to accurately rank miners’ output to obtain higher VTRUST scores and increase their TAO rewards to form a network consensus mechanism.
Sub -network and application
The interaction on Bittersor mainly includes the verifications submitted requests to the miners and evaluated their output.However, with the improvement of the quality of miners and the growth of the overall intelligence of the network, Bittensor will create an application layer on its existing stack so that developers can build applications that query Bittensor networks.
In October 2023, Bittensor introduced subnets through Revolution upgrades and took an important step towards achieving this goal.Ziwang is a separate network that inspires specific behaviors on Bittersor.Revolution opens the network to anyone who is interested in creating a subnet.Within a few months since its release, more than 32 sub -nets have been started, including subnets for text prompts, data capture, image generation and storage.With the maturity of the subnet and becoming ready, the creator of the subnet will also create an application integration so that the team can build an application for querying specific subnets.Some applications (chat robots, image generators, Twitter response robots, predictive markets) already exist, but except for the funding of the Bittersor Foundation, there are no formal incentive measures for verifications to accept and forward these queries.
In order to provide a clearer explanation, the following is an example, explaining how the Bittersor may work after the application is integrated into the network.
The performance of the subnet is based on the performance of the root network.The root network is located above all subnets. It essentially acts as a special subnet and is managed by 64 largest subnet verifications according to equity.The root network verification persons rank the subnet based on the performance of the subnet, and regularly distribute the emitted TAO token to the subnet.In this way, each sub -network acts as miners of the root network.
Bittersor Outlook
Bittersor is still experiencing the trouble of growth, because it expands the function of the protocol to motivate the intelligent generation of multi -sub -nets.Miners continue to design new methods to attack the network to get more TAO rewards, such as the output of high evaluation and reasoning by modifying its model operation, and then submit multiple variants.The governance proposal affecting the entire network can only be submitted and implemented by Triumvirate, which is completely composed of the interest -related interest of the OpenEnsor Foundation (it should be noted that the proposal needs to be approved by the Bittensor Senate composed of Bittensor verified by the Bittensor).The token economy of the project is being amended to increase the incentives for the use of TAO cross -child network.The project also quickly became famous due to its unique method. The CEO of HuggingFace, one of the most popular artificial intelligence websites, said Bittersor should add its resources to the website.
In an article recently published by the core developer, in an article entitled “Bittensor Paradigm”, the team explained the vision of Bittersor, that is, eventually developed into “unknown content measured”.Theoretically, this allows Bittensor to develop subnets to motivate all types of behaviors supported by TAO.There are still considerable actual restrictions -the most noteworthy is that these networks can expand to deal with such a diverse process, and the progress of potential incentive measures has exceeded centralized products.
Build a decentralized calculation stack for the AI model
The above parts provide a rough overview of various types of decentralized AI computing protocols that are developing.In the early days they developed and adopted, they provided the foundation of the ecosystem, and ultimately promoted the creation of “AI build blocks”, such as the concept of “Monetary Lego” of Defi.The combined availability of the blockchain without permission provides the possibility for each protocol to build a more comprehensive decentralized artificial intelligence ecosystem.
For example, this is a way that Akash, Gensyn and Bittensor may interact to respond to reasoning requests.
What needs to be clear is that this is just an example of what may happen in the future, not the representative of the current ecosystem, existing partnerships or possible results.Mutual operational restrictions and other considerations described below have greatly limited the possibility of today’s integration.In addition, the needs of liquidity and the need to use multiple tokens may damage the user experience. Both the founders of Akash and Bittersor pointed out this.
Other decentralized products
In addition to calculation, several other decentralized infrastructure services have been launched to support the emerging AI ecosystem of cryptocurrencies.
List all the scope of this report, but some interesting and explanatory examples include:
-
Ocean:A decentralized data market.Users can create data NFT representing their data and can use data tokens for purchases.Users can monetize their data and have greater sovereignty, and at the same time provide access to the AI team with access to the data required by the development and training models.
-
Grass:A decentralized bandwidth market.Users can sell excess bandwidth to AI, and the latter uses these bandwidth to capture data from the Internet.Grass is based on the WYND network, which not only enables individuals to monetize their bandwidth, but also provide more diverse views for bandwidth buyers to understand the content that individuals see on the Internet (because personal Internet access usually usuallyIt is customized according to its IP address)).
-
Hivemapper:Build a decentralized map product, which includes information collected from daily car drivers.HiveMapper relies on AI to explain images collected from the user instrument board camera, and reward users to help fine -tune the AI model to tokens by strengthening human learning feedback (RHLF).
Overall, these point to the almost infinite opportunity to explore the decentralized market models that support AI models or develop their required peripheral infrastructure.At present, most of these projects are in the conceptual verification stage, and more research and development requires that they can operate the scale required for comprehensive artificial intelligence services.
Look forward to
Decentralized computing products are still in the early stages of development.They have just begun to launch the most advanced computing power that can train the most powerful AI model in production.In order to obtain meaningful market share, they need to show the actual advantages compared with centralized alternatives.The potential trigger factor more widely adopted includes:
-
GPU supply/demand.The scarcity of GPU and the rapid growth of calculation are leading to the GPU military reserve competition.Due to the limitation of the GPU, Openai has restricted access to its platform.Platforms such as Akash and Gensyn can provide cost -effective alternatives for teams that need high -performance computing.For decentralized computing providers, the next 6-12 months will be a particularly unique opportunity to attract new users. Due to the lack of wider market access, these new users are forced to consider decentralized products.Coupled with the increasing open source models such as META’s LLAMA 2, users no longer face the same obstacles when deploying effective fine -tuning models, making computing resources the main bottleneck.However, the existence of the platform itself does not ensure sufficient computing supply and corresponding needs of consumers.It is still difficult to purchase high -end GPUs, and the cost is not always the main motivation for the demand side.These platforms will face challenges to show the actual benefits of using decentralized calculation options (whether due to cost, review resistance, normal operation time and elasticity or accessibility) to accumulate viscosity users.They must act quickly.GPU infrastructure investment and construction are carried out at an alarming rate.
-
Supervision.Supervision is still the resistance of decentralized calculation movement.In the short term, lack of clear supervision means that providers and users are facing potential risks to use these services.What if the supplier provides calculations or the buyer is calculated from the sanction entity without knowing it?Users may hesitate whether to use decentralized platforms that lack centralized entity control and supervision.The agreement tries to reduce these concerns by incorporating control into its platform or adding a filter to only access the known computing provider (that is, providing your customer KYC information), but more powerful methods need to ensure compliance while protecting the compliance while protecting the compliance.privacy.In the short term, we may see the emergence of the KYC and compliance platforms. These platforms restrict the access to their protocols to solve these problems.In addition, the discussion on possible new regulatory frameworks in the United States (the best example is the release of administrative orders for security, reliability and trustworthy artificial intelligence development and use) highlights the potential of further restricting the GPU acquisition of regulatory actions.
-
Examine.Regulatory is two -way, decentralized computing products can benefit from the actions restricting AI access.In addition to administrative orders, OPENAI founder Sam Altman also testified in Congress, indicating that regulators need to issue licenses for artificial intelligence development.The discussion on artificial intelligence supervision has just begun, but any such restrictions to access or review AI functions may accelerate the use of decentralized platforms without such obstacles.In November 2023, the changing (or lack of) the leadership of the OpenAI leadership was further stated that it was risky to grant the decision -making power of the most powerful AI model to a few people.In addition, all AI models will inevitably reflect the prejudice of people who create them, whether intentional or unintentional.One way to eliminate these deviations is to make fine -tuning and training as much as possible to ensure that anyone in any place can access various types and deviation models.
-
Data privacy.When the external data and privacy solutions that provide users with data autonomy are integrated, decentralization calculations may be more attractive than centralized alternatives.When Samsung realized that the engineer was using ChatGPT to help the chip design and leaked sensitive information to ChatGPT, Samsung became the victim of the incident.Phala Network and IEXEC claim to provide users with SGX secure land to protect user data, and the ongoing full -state encryption research can further unlock the decentralized calculation of privacy to ensure privacy.As AI further integrates into our lives, users will pay more attention to running models on applications with privacy protection.Users also need to support a combined service of data so that they can transplant data from one model to another model.
-
User experience (UX)EssenceUser experience is still a major obstacle to all types of encrypted applications and infrastructure.This is not different for decentralized computing products, and in some cases, because developers need to understand cryptocurrency and artificial intelligence, this will exacerbate this situation.It is necessary to improve from basic knowledge, such as logging in abstraction with the interaction with the blockchain to provide the same high -quality output as the current market leaders.Given that many operating decentralized calculation protocols that provide cheaper products are difficult to obtain conventional use, this is obvious.
Smart contract and zkml
Intelligent contracts are the core construction blocks of any blockchain ecosystem.In the case of a set of specific conditions, they will automatically execute and reduce or eliminate the needs of trusted third parties to create complex decentralized applications, such as applications in DEFI.However, because most of the smart contracts currently exist, their functions are still limited because they are executed based on the preset parameters that must be updated.
For example, the deployed lending agreement intelligent contract contains the specifications of when to clear the position according to the specific loan to the value ratio.Although it is useful in a static environment, under the dynamic situation of risks, these smart contracts must be continuously updated to adapt to changes in risk tolerance, which brings challenges to contracts that do not pass the centralized process management.For example, DAOs that rely on decentralized governance processes may not be able to quickly respond to systemic risks.
Integrated AI (that is, machine learning model) smart contracts are a possible way to enhance functions, security and efficiency while improving the overall user experience.However, these integrations also bring additional risks, because it is impossible to ensure that the models that support these smart contracts will not be attacked or explained to the long tail situation (in view of the scarcity of data input, the long tail situation is difficult to train the model).
Zero -knowledge machine learning (zkml)
Machine learning requires a lot of calculations to run complex models, which makes the AI model cannot operate directly in smart contracts due to high cost.For example, the DEFI protocol that provides users with an income optimization model will be difficult to run the model on the chain without paying too high GAS fees.A solution is to increase the computing power of the underlying blockchain.However, this also increases the requirements for the album of chain verification, which may destroy decentralized characteristics.Instead, some projects are exploring that ZKML is used to verify the output without trust, and does not require dense chain calculations.
It shows that a common example of ZKML’s usefulness is that users need other people to run data through the model and verify that their transactions have actually run the correct model.Perhaps developers are using decentralized computing providers to train their models, and worry that the provider tries to cut costs by trying to use the output differences that can hardly detect.ZKML enables the computing provider to run data through its model, and then generates proves that can be verified on the chain to prove that the output of the given input model is correct.In this case, the model provider will have additional advantages, that is, they can provide their models without having to disclose the basic weight of the output.
You can also do the opposite.If the user wants to use their data to run the model, but because of privacy issues (such as medical examinations or proprietary business information), do not want to provide models to provide models to access their data, then users can run on their dataThe model does not share the data, and then proves that they run the correct model by verifying them.These possibilities have greatly expanded the design space of artificial intelligence and smart contract functions by solving the discouraged calculation restrictions.
Infrastructure and tools
In view of the early state of the ZKML field, the development is mainly concentrated in the infrastructure and tools required by the construction team to convert its models and output into proofs that can be verified on the chain.These products abstract as far as possible zero knowledge.
Ezkl and gizaIt is the two items that build this tool by providing verification proof of machine learning models.Both help the team to build a machine learning model to ensure that these models can be executed in a trusted manner on the chain.Both projects use the open neural network switch (onnx) to convert machine learning models written by common language such as TensorFlow and PyTorch into standard formats.They then output the versions of these models, which will also generate ZK proof during execution.EZKL is open source, producing ZK-SNARKS, and GIZA is closed, producing ZK-Starks.These two projects are currently only compatible with EVM.
In the past few months, EZKL has made significant progress in enhancing ZKML solutions, mainly focusing on reducing costs, improving safety, and accelerating proof of generating generation.For example, in November 2023, EZKL integrated a new open source GPU library that shortened the aggregate certificate time by 35%; in January, EZKL released Lilith, which is a software solution for integration when using EZKL proof to integrate when using EZKL proof.High -performance computing cluster and arrangement system.The uniqueness of GIZA is that in addition to providing tools for creating verified machine learning models, they also plan to realize Web3 equivalent to Hugging Face, open up the user market for ZKML collaboration and model sharing, and eventually integrate decentralized decentralizationCalculate the product.In January, EZKL released a benchmark evaluation that compared the performance of EZKL, GIZA and Risczero (as described below).EZKL shows faster proof time and memory use.
Modulus LabsIt is also developing a new ZK-PROOF technology customized for the AI model.Modulus published a paper called “The Cost of Intelligence” (suggesting that the cost of running the AI model on the chain was extremely high).To determine the ability and bottleneck of improving the AI model ZK-Proofs.The paper was published in January 2023, indicating that existing products are too expensive and inefficient to achieve AI applications on a large scale.On the basis of initial research, Modulus launched Remainder in November, which is a specialized zero -knowledge certificate that is specifically used to reduce the cost and certificate of the AI model.Integrated into smart contracts.Their work is closed, so they cannot test the benchmark with the above solutions, but recently quoted their work in Vitalik’s blog post on encryption and artificial intelligence.
The development of tools and infrastructure is important for the future growth of the ZKML space, because it can significantly reduce the friction of the team required to deploy the verified chain to calculate the required ZK circuit.Create a security interface to enable non -encrypted native builders engaged in machine learning work to bring their models to the chain, which will enable applications to perform larger experiments through truly novel use cases.The tool also solves a major obstacle to the use of ZKML, that is, developers who are interested in working in the field of cross -knowledge of zero knowledge, machine learning and cryptography.
COPROCESSORS
Other solutions (referred to as “Synthetic Processor”) are developing.Risczero, axiom, and ritualEssenceThe term of the collaborator is mainly semantically -these networks perform many different roles, including calculation under the verification chain on the chain.Like Ezkl, Giza and Modulus, their goal is to fully abstract zero -knowledge proof of the generation process. Create a zero -knowledge virtual machine that can perform the programs under the chain and generate the provenance of the chain.Risczero and Axiom can provide services to simple AI models because they are more common collaborators, and Ritual is specially built for use with the AI model.
InfernetIt is the first instance of Ritual, which contains an INFERNET SDK, allowing developers to submit reasoning requests to the network and receive output and proof (optional) as a return.Infernet nodes receive these requests and calculate under the processing chain before returning output.For example, DAO can create a process to ensure that all new governance proposals meet certain prerequisites before submitting.Each time a new proposal is submitted, the governance contract will trigger the inference request through the Infernet to call the AI model of DAO specific governance training.The model review proposal to ensure that all necessary standards are submitted, and the output and evidence are returned to the submission of the proposal.
In the next year, the Ritual team plans to launch more functions to form infrastructure layers called the Ritual Super Chain.Many of the items discussed earlier can be inserted into Ritual as a service provider.The Ritual team has integrated with Ezkl to generate proof, and may soon add the functions of other leading providers.Infernet nodes on Ritual can also use Akash or IO.NET GPUs and query models trained online in Bittersor sub -online.Their ultimate goal is to be the first choice provider of open AI infrastructure, which can provide services for machine learning and other AI related tasks for any network and any workload.
application
ZKML helps to reconcile the contradiction between blockchain and artificial intelligence. The former is essentially limited resources, while the latter needs a lot of calculations and data.As a founder of Giza said, “The use case is very rich … this is a bit like the use case of Ethereum in the early days of Ethereum … What we have done is only the use case of expanding smart contracts.” However, as mentioned above, today, todayDevelopment mainly occurs in tools and infrastructure levels.The application is still in the exploration stage. The challenge facing the team is to prove that the value generated by the use of ZKML to implement the model exceeds its complexity and cost.
Some of the current applications include:
-
Decentralized financeEssenceZKML has upgraded the design space of DEFI by enhancing the ability of smart contracts.The DEFI protocol provides a large amount of verified and non -tampered data for machine learning models, which can be used to generate income acquisition or trading strategies, risk analysis, user experience, etc.For example,Giza andYearn FinanceCooperate to build a concept verification automatic risk assessment engine for Yearn’s new V3 vault.Modulus Labs cooperates with Lyra Finance to incorporate machine learning into its AMM, withIon ProtocolCooperate implementing the model of verification and verifier risk, and helpUpshotNFT price information that verifies its artificial intelligence.Noya(Using Ezkl) and Mozaic agreements provide access to the proprietary chain models. These models allow users to access automated liquidity mining, and at the same time enable them to verify the data input and proof of data on the chain.Spectral financeThe credit scoring engine on the chain is constructing the possibility of the ownership of Compound or AAVE borrower in arrears.Due to ZKML, these so-called “DE-AI-Fi” products may become more popular in the next few years.
-
gameEssenceFor a long time, the game has been considered to be subverted and enhanced through the public chain.ZKML is possible to make artificial intelligence chain games.Modulus Labs has realized the concept verification of the simple chain game.Leela vs the worldIt is a gaming theory of chess games. Users are fighting AI chess models in it. Every step that ZKML verifications Leela are based on game operation.Similarly, the team also uses the EZKL framework to build a simple singing competition and chain of the game.CartridgeGiza is using GIZA to enable the team to deploy a full chain game. Recently, a simple artificial intelligence driving game has been introduced. Users can compete for a better model for cars trying to avoid obstacles.Although simple, these concept verification points to the future implementation, which can achieve more complex chain verification, such as complex NPC actors that can interact with the economy in the game, such as “Ai ArenaWhat you see in the “see, this is a super -chaotic game. Players can train their soldiers in them, and then deploy to fight for the AI model.
-
Identity, traceability and privacyEssenceCryptocurrencies have been used to verify authenticity and cracking down on more and more artificial intelligence generation/manipulation content and in -depth falsification.ZKML can advance these efforts.WorlddcoinIt is an identity proof solution that requires users to scan the iris to generate unique IDs.In the future, biometric IDs can be stored on personal devices for self -hosting, and the models required to verify local biometrics are used to verify local biometrics.Users can then provide evidence of biological identification without revealing their identity, so as to ensure privacy while resisting the witch attack.This can also be applied to other inferences that require privacy, such as using models to analyze medical data/images to detect diseases, verify personality, and develop algorithms in dating applications, or insurance and loan institutions that need to verify financial information.
Look forward to
ZKML is still in the experimental stage, and most projects focus on the construction of infrastructure’s original terms and concept proof.Today’s challenges include calculation costs, memory restrictions, model complexity, limited tools and infrastructure, and developers.In short, before ZKML was implemented by the scale required by consumer products, there were quite a lot of work to do.
However, with the maturity of this field and the resolution of these restrictions, ZKML will become a key component of AI and encryption.In essence, ZKML promises to calculate the chain of any size into the chain, while maintaining the same or close security assurance as the chain.However, before the realization of this wish, the early users of the technology will continue to weigh between the privacy and security of ZKML and the efficiency of alternatives.
AI Smart
One of the most exciting integrations of AI and cryptocurrencies is the ongoing AI smart experiment.Intelligence is an autonomous robot that can receive, interpret and execute tasks in AI models.This can be anything, from a personal assistant to fine -tuning based on your preferences to hire a financial robot that manages and adjust your investment portfolio based on your risk preferences.
Because cryptocurrencies provide payment infrastructure without permission and no trust, smart and cryptocurrencies can be combined well.After training, the smart body will get a wallet so that they can use smart contracts for transactions by themselves.For example, today’s simple intelligence can capture information on the Internet, and then trade in the forecast market according to the model.
Smart provider
MorpheusIt is one of the latest open source smart projects listed on Ethereum and Arbitrum in 2024.Its white paper was anonymous in September 2023, providing the foundation for the formation and construction of the community (including famous characters such as Erik Vorhees).The white paper includes a downloadable smart protocol. It is an open source LLM that can run locally, manages the user’s wallet, and interacts with smart contracts.It uses smart contract rankings to help smartists determine which smart contracts can be safely interacting based on standards such as transactions.
The White Paper also provides a framework for building an Morpheus network, such as the incentive structure and infrastructure required for the operation of the smart protocol.This includes the front -end of the incentive contributor to build an API that can be inserted into an application that can interact with each other in the front end of interaction with smart bodies, and the cloud that can interact with each other.The solution is on the edge device.The initial funds of the project have been launched in early February, and the full agreement is expected to start in the second quarter of 2024.
Decentralized autonomous infrastructure network (DAIN)It is a new intelligent infrastructure agreement that builds an intelligent physical economy on Solana.The goal of DAIN is to allow intelligence from different enterprises to interact seamlessly through common APIs, so as to greatly open the design space of AI smart parties, the focus is to realize smart parties that can interact with Web2 and Web3 products.In January, DAIN announced the first cooperation with Asset Shield. Users can add “smart signatures” to their multiple signatures. These signatures can explain the transaction and approve/reject according to the rules set by the user.
Fetch.aiIt was one of the earliest AI smart parties that deployed and developed an ecosystem to build, deploy and use smart bodies on the chain using FET tokens and fetch.ai wallets.The protocol provides a set of comprehensive tools and applications for using smart bodies, including in -wallet functions for interaction with smart bodies and ordering agents.
AutonolasThe founder includes the former member of the FETCH team, which is an open market for creating and using decentralized AI smartmen.Autonolas also provides developers with a set of tools to build AI smart parties under the chain, and can insert multiple blockchains, including Polygon, Ethereum, GNOSIS Chain and Solana.They currently have some active smart concept verification products, including prediction of markets and DAO governance.
SingularityNetIt is building a decentralized market for AI Smart, and people can deploy focused AI smart parties in them. These intelligences can be hired by others or smart parties to perform complex tasks.Other companies, such as AlteredStateMachine, are constructing the integration of AI smart and NFT.Users cast NFT with random attributes, which give them the advantages and disadvantages of different tasks.These intelligence can be trained to enhance certain attributes to be used for games, DEFI or as virtual assistants and trade with other users.
In general, these projects imagine a future smart ecosystem. These intelligences can work together, not only can perform tasks, but also help build universal AI.The truly complicated intelligence will be capable of completing any user tasks independently.For example, a complete autonomous intelligence will be able to figure out how to hire another smart body to integrate the API, and then execute it without having to ensure that the smart body has integrated and executed the task with the external API (such as a travel booking website) before use.From a user perspective, you do not need to check whether the smart body can complete the task, because the smart body can determine itself.
Bitcoin and AI Smart
July 2023,Lightning Network LabThe concept verification embodiment of the use of smart body on the Lightning Network was launched, which is calledLANGChain Bitcoin KitEssenceThis product is particularly interesting because it aims to solve the increasingly serious problem in the world of web 2 -the access control and expensive API key of the WEB application.
Langchain solves this problem by providing a set of tools for developers, enabling the intelligence to buy, sell and hold Bitcoin, and query the API key and send small payment.In the traditional payment field, small payment is high due to expenses. On the Lightning Network, smart parties can send unlimited small payment at the lowest cost every day.When combined with Langchain’s L402 payment measurement API framework, this allows the company to adjust the access cost of its API according to the increase and reduction of the usage, rather than set a standard for a single cost.
In the future, the on -chain activity is mainly led by the interaction of smart body and smart body. Such things will be necessary to ensure that the intelligent body can interact with each other in a high cost.This is an early example that shows how to use intelligence on the track without licensed and economic efficient payment, which has opened up the possibility of new markets and economic interaction.
Look forward to
The intelligent field is still in the new stage.The project has just begun to launch functional intelligence, which can use its infrastructure to handle simple tasks -this is usually only experienced developers and users.However, over time, one of the biggest impacts of AI intelligence on cryptocurrencies is the improvement of user experience in all vertical fields.The transaction will begin to turn from clicks to text, and users can interact with the intelligent body on the chain through large language modulus.Dawn Wallet et al.The team has launched a chat robot wallet for users to interact on the chain.
In addition, it is unclear how smart parties operate in Web 2, because the financial sector depends on regulated banking institutions, these institutions cannot operate 24/7 and cannot conduct seamless cross -border transactions.As Lyn Alden emphasizes, due to the lack of the ability to refund and processing micro -transactions, the crypto track is particularly attractive compared to credit cards.However, if the intelligence becomes a more common method of transaction, existing payment providers and applications are likely to take action quickly to implement the infrastructure required for operating in the existing financial field, thereby weakening some of the use of cryptocurrenciesbenefit.
At present, intelligence may be limited to cryptocurrency transactions, of which given input guarantee given output.Both models specify these intelligent body to figure out how to perform complex tasks, and the tools expand the range they can complete, and both need to be further developed.In order to make the encrypted intelligence be useful on the new chain encryption use cases, more extensive integration and acceptance of encryption are needed as a form of payment and the clarification of supervision.However, with the development of these components, the intelligent body is preparing to become one of the largest consumer of the above -mentioned decentralized computing and the ZKML solution, receiving and resolving any tasks in an autonomous non -confirmed way.
in conclusion
AI introduces the same innovation we see in Web2 for cryptocurrencies, and enhance all aspects from infrastructure development to user experience and accessibility.However, the project is still in the early stage of development, and recent cryptocurrency and AI integration will be mainly led by the under -chain.
Products such as Copilot will “increase by 10 times” developers. Layer1 and DEFI applications have cooperated with large companies such as Microsoft to launch an artificial intelligence -assisted development platform.Companies such as CUB3.AI and Test Machine are developing AI integration for smart contract audits and real -time threat monitoring to enhance the security of the chain.LLM chat robots are training on chain data, protocol documents and applications to provide users with enhanced accessability and user experience.
For the more advanced integration of the use of cryptocurrency underlying technology, challenges still prove that the implementation of AI solutions on the chain is technically feasible, and it is also economical.The development of decentralized calculations, ZKML, and AI intelligence points to the prospective vertical fields, which laid the foundation for the future of cryptocurrencies and AI in -depth interconnection.